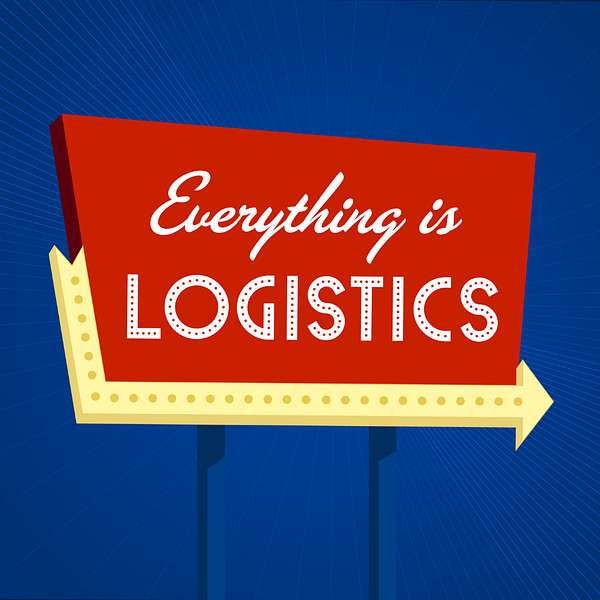
Everything is Logistics
A podcast for the thinkers in freight. Everything is Logistics is hosted by Blythe Brumleve and we're telling the stories behind how your favorite stuff (and people!) get from point A to B.
Industry topics include freight, logistics, transportation, maritime, warehousing, intermodal, and trucking along with the intersection of technology and marketing within the industry.
123k downloads and rated as a top 5% podcast out of all industries and growing. Follow along to stay curious and become a better thinker in freight.
Everything is Logistics
Freight Tech Unveiled: Innovations Transforming Logistics
Get a crash course in the tech transforming global supply chains, from warehouse drones to AI-powered optimization, with three can't-miss conversations from industry innovators.
Metafora’s Chief Growth Officer Ryan Schreiber dives into the realities of AI implementation, LoadPartner Co-founder Garrett Allen breaks down what AI means for logistics teams, and Gnosis Freight CTO Jake Hoffman reveals how better visibility is reshaping container shipping. Their expert insights cut through the hype to uncover where freight tech is really headed.
LINKS:
- Advancing Freight Tech with Ryan Schreiber
- A Crash Course in Freight AI with Garrett Allen
- The Container Operating System with Gnosis Freight
Feedback? Ideas for a future episode? Shoot us a text here to let us know.
-----------------------------------------
THANK YOU TO OUR SPONSORS!
Are you experienced in freight sales or already an independent freight agent? Listen to our Freight Agent Trenches interview series powered by SPI Logistics to hear from the company's agents on how they took the leap and found a home with SPI freight agent program.
CloneOps AI-powered phone operations for inbound and outbound calls with speed, scale, and efficiency. Our virtual agents handle high-volume interactions, automate workflows, and deliver real-time insights, freeing your team to focus on growth. Designed for logistics, retail, and beyond—seamless communication, smarter conversations, faster resolutions.
CargoRex – Your Logistics Hub. Explore, discover, and evolve with the all-in-one platform connecting you to the top logistics tools, services, and industry voices. Whether you're a leader, researcher, or creator, CargoRex helps you stay ahead. Explore Now
Digital Dispatch maximizes your #1 sales tool with a website that establishes trust and builds rock-solid relationships with your leads and customers. Check out our website services her...
Music.
Blythe Brumleve:Welcome into a very special edition of everything's logistics, where we're highlighting some of your favorite podcast episodes in 2024 covering all things technology. Think of this as a super episode, where three of our most popular technology subject matter experts. They're all in one episode, so you don't have to go digging around for the good stuff. If you don't know me, my name is Blythe, and our show for covers topics for the thinkers in freight to help tell the stories of how your favorite stuff and people make it from point A to B. So for this episode, we have three freight tech topics back to back. First one being advancing freight Tech with Ryan Shriver. The next one, we have a crash course in freight. Ai with Garrett Allen, and we round out the show with the container operating system with Genesis freight. All of these episodes experience the most plays in the technology category for the podcast. So in case you missed it or simply want to refresh. I got you covered. Each episode will play after each other with the fancy little whoosh sound effect to signify the start of the next episode. I've also got timestamps in the show notes, in case you would like to skip around with all that said, cheers to 2025 thanks for all the love and support for the podcast, and I hope you all enjoy this best of Roundup. Welcome
Unknown:into another episode of everything is logistics, a podcast for the thinkers and freight. We are proudly presented by SPI logistics, and I am your host, Blythe Brumleve. We've got another amazing guest for you today, and this conversation is already off the rails, and we just started recording, but we just started recording, so I wanted to make sure that I give you something as to celebrate your fandom, Jaguars. This looks used, which I really appreciate. So like, was this? Was this like a game day turmoil? Got it experienced by that. So I thought you would be honest with you, I don't know what type of experience happens in Jacksonville, but I know that. I probably don't want my hands on it, but I have emotional trauma. It's the thought that counts. Yeah, also a lot of urine, probably, but,
Ryan Schrieber:yeah, I mean, I hope, but definitely alcohol sweat, that's yeah, for sure, but it's the thought. Thank you very much. I appreciate it. I will use this on my I don't really drink, so I don't know, actually, when I'm even going to use this. I'll use it on my your water bottles. Yeah, there you go. Water bottles. And now, for folks who are just listening, Ryan is a huge Jaguars fan. We connected about we bonded over the Jaguars. He's actually a Tampa fan, which just crucified my team last season. So we haven't really talked about the team talked about the Jaguars since then. So it's a little bit of a sore subject, but we share that people when they're down, I like to kick them when they're up, you know, so and there's like, remind them of how, kind of, like, keep people grounded in the fact that you like, you know, you're still the Jaguars. I know it's a realization. It's sort of the picks and valleys of being a fan. So you have to appreciate the wins when you got them, because, you know, they're not going to be very some good years, but they've lot of really bad my first, like, football memory was, you know, it's probably like 1994 it was opening day at the old stadium, and the bucks were playing the Kansas City Chiefs and i So, if it was 1994 I was 10, you know, and it was, Like, unbelievably hot. Like, you know, they had a metal seats in the old stadium. So I get it, it's a lot of down years. And, you know, Florida sports teams in general, oh, God, God. Who are you telling except for the Gators? They had, you know, very successful run, begrudgingly, yeah. But very lot of down times recently, like, they already want to fire this guy with their coaches. Thank God. That's I just wish nothing but suffering to that fan base.
Unknown:I don't know that. I've actually said who is, you know, who we're talking to right now. We're right Shriver. I'm Ryan. Yeah, you work for metaphor. Are you co founder or founder? What's your what's your role with I'm the chief growth officer for metaphor. So metaphor is we do technology consulting, but we focus exclusively on transportation, logistics, supply chain. So you know, more or less, we help our customers figure out what to do with technology in their business. We and then we help them execute on on whatever that strategy might be, technology and data, and then execute on that strategy whatever it might be. We help them procure software. We help them implement software. We help them build software. So that's a little bit of what we do. How did you what? What did you do before? Metaphor, so I come from the industry. I come I started my career at Echo global logistics about 15 years ago, just as a freight broker slinging freight, I started a few companies in the space. So I started a couple legacy brokerages. I started my own invented consulting business at one point, which was a huge failure, just like unbelievable failure. How so? Well, I couldn't really get customer, like, I couldn't pay my bills, basically, you know, and it was like I really struggled with, like getting customers and also, like trying to deliver solutions for customers. I learned a really valuable lesson that I was also a little half in half out, because, like, I knew somebody would hire me and pay me to a lot. Money to, you know, build their brokerage, or run their brokerage, or or what have you. And I so I didn't give my all to the thing I kind of like, was half in, half out, trying to figure out, you know, maybe trying to also find a job. And so I kind of learned a really valuable lesson there about, like, commit, being committed to the to, like, the thing that you're trying to, especially if you're trying to start something from scratch. Even though I had started two businesses from scratch before, scratch before then, I started a tech company. We started in 2016 we were a digital brokerage. We were applying generative AI. We didn't call it that back then, but we were applying generative AI instead of an app to do digital logistics. And then I've been with metaphor for about five years. So I come from industry like I, that's my background is, you know, the space is not being logistics, I guess, what was the catalyst of joining metaphor? So, I that's a really, what was the catalyst? So one other thing you learn when you do kind of startups, if you will, especially if you know, when you have, you get, you're getting into bed with with people, and if you raise capital from folks, like you're getting into bed with your capital providers, if you have co founders, like you're getting married to these folks. And so I was the freight guy, and my two other co founders were more tech folks, and we just had some very, very fundamental disagreements about strategy. And so I decided to move on from the business, and unfortunately, like we the business failed as, not necessarily as a result, but also not, not as a result. And so I was trying to find the next thing that I wanted to do, and and it was that was really hard for me. I hate brokerage. I hate trucking. I just, I hate them in the sense that they're very broken businesses. The operating models are broken, and they really break people. I mean, they really do. And so I spent some time thinking about like, what do I need, Adam, the next opportunity? Like, what I know, what I bring to the table? Like, what do I need to get back from a company? And so I spent a lot of time thinking about that. I had a couple of opportunities, you know, that kind of was picking from but metaphor really, like, it was a great team, still a great team, a great problem. Like, I'm a very curious person, so I like being able to solve different problems. And so it gave me the opportunity. Consulting gives you the opportunity to see different problems, see them manifest themselves differently. But also, like, move from, you know, move to, from problems to problems. And so gave an interesting vantage point. I thought it would, I thought it would give me an intellectually stimulating experience, which it has. So like, I don't know that answered the question. No, it does. I'm curious, though, what are some of those big problems that you're solving, or maybe exciting, big problems that you're solving today, some of the really interesting, like, you know, there everybody, I think it's human nature to trend chase a little bit. And so, of course, like all of our conversations over the last 12 months, the conversation, all of them somehow touch on artificial intelligence, machine learning. Not that we weren't having those conversations before, but they certainly accelerated. And so some really interesting challenges when you think about the space of transportation logistics. So our customers are our shippers. They're transportation providers, they're freight technology companies. Their private equity that serve the space of trucking and logistics and supply chain, and so data is a big problem. So AI readiness, like most there are areas where you can apply AI today, generative, some generative AI use cases around natural language processing can deliver value. But the reality is, most companies don't have the data strategy. People talk about clean data. That's a thing for sure. It starts with actually, like getting all of the data together that you need. When I walk into these businesses, a lot of times I look around and I just see all the data. That's like lying on the ground. So as an example, like most folks really, really underestimate the value of of the data from phone calls. And I don't mean what people are saying, but being able to, like, see who's calling, sorry excuse me, like, but to be able to tie together things like, you know, this phone number is associated with this driver that's associated with this carrier that's on this shipment, and what was happening with that shipment when that driver called in. So giving context to data and so, you know, there's a lot you can learn about the behavior, what's kind of referred to as observed behavior of participants, and you need a really connected technology ecosystem, and you need a connected data strategy to kind of unlock some of those things. So AI readiness is a really interesting problem that we that we work on these days. What are some tips, I guess, for companies to maybe want to, you know, are thinking about that next step and thinking about, you know, they've had aI shoved down their throat for the last year, totally. So how are they, how should they prepare, I guess, for AI or data ready? Yeah, focus on integrations. Like, focus on, well, let me rephrase that, focus on getting access to the data that you have. And then step one is, for the last 10 or 15 years, we've really focused on, like, get your hands on data and put it in front of a user. Okay? Well, users have too much data to parse. Humans can't consume the amount of data that we're talking about. So in the absence of a clear path forward, they make things up like genuinely like these, because there's enough data to validate or justify the decision. On the back end, you can point to these data points supposed to these data points. So step one, focus on getting access to as much different types of data as you can your phone like, you know, phone information. A lot of vendors, especially if you have, like, off the shelf things, they have tools that underlie analytics, tools that maybe you're not using to but then make sure that those are connected, and then, and then focus on a data strategy. So like, how are you master data? Management is a term. So focus on how you're managing your data, where the data is moving, and then how you can get access to that data, how that data is structured. Those are all like, they're obviously difficult and thorny problems, but AI and machine learning are very difficult things to do. It's not as simple as saying, like, Hey, okay, we're going to do, we're going to do AI and and so there are certainly vendors that can help you with that. There are off the shelf tools that are making use of AI. Be careful with those, like some folks are talking about AI, that aren't doing AI but, but also really getting access to that data, and then the most important thing is answer the question, what do you want to do? What are the use cases? Ai ml, exactly. AI ML is not some like magic elixir, like, what are the questions you want answered to insight into so one of our large customers I've been talking to, you know, they have a support staff. You know that's doing, you know, kind of like shared services around, you know, answering inbound phone calls for everything from, like, what's my load number, or, excuse me, what's my delivery number to what's my payment status. Well, like analyzing those phone calls to understand what's the most common problems these people are solving, so that you can then automate from from there. So, so really focus on, on like, what are the business problems that you want to solve with technology? That's true of AI machine learning. That's just true of anywhere, anywhere you're going to apply technology. What's the, what's, what's the business problem I'm solving, what's the question that I want to get answered? And then, and then, you know, obviously get get access to that data. Are there any questions that they should be asking? Maybe they don't know the questions that they should be asking of their data. Is there maybe, like, a framework that you work with your clients with, they should probably ask themselves, like, how do they free up money to work with somebody like me? But you know what a framework to work with the data. Yes, that's a really like, I don't know that I got enough time to really walk through a framework, especially without some some visuals. But, you know, I would say that really the key, kind of, like, one of the keys is, is, is making sure that the data is like the Master Data Management, having a cohesive and consistent kind of, like way of of describing a piece of of datum. So, you know, like as an example, you know, how, how was a shipment conceptualized from a data perspective, within the entire ecosystem and so. But there's no one size fits all answer for any of this. Like, every business is discrete. There are certainly like ways in which these businesses are similar. Otherwise, you know, we wouldn't be able to do anything together, or there wouldn't be a use of technology, but, but that, you know, if you're, if you're, if you're a traditional three PL doing distribution versus your shipper, you know, doing chemical versus healthcare, or you're, like, a freight broker or trucking company that's doing, you know, last mile. How you think about pieces of data that's going to differ by your business? And so there's no kind of, like, one size fits all answer for how to look at that, but, but yes, I mean, I think if you need, if these are things that you really want to do educate yourself on, you know, data management, and it doesn't require, there's, there's ways to make incremental improvement that aren't millions of dollars of investment. And so certainly, like, that's, that's a way to, kind of like, start to think about it, and a lot of it will start with kind of what your technology ecosystem looks like and what tools you have, and how they're helping you get access to the underlying data and how easy they are to tie together with other things. One thing that's really helped change a lot of my my marketing is using these AI No. Taking apps that automatically join the meeting, transcribe it, and then I'm able to filter through and parse through a lot of that data with different customers. Yeah, can a lot of three PLS, or carriers, maybe tech companies, can they utilize a similar approach with some of these tools? Yeah? So, like, that's leveraging natural language processing. And so like, again, my last business was using natural language processing to digitize, like, a lot of things within a brokerage, and absolutely like NLP, especially because, like, you're not going to build, you know, NLP is built on natural language processing. NLP is built on large language models. That's what we refer to as deep tech. You're not going to build a new large language model. And the great thing about, you know, where we are today with NLP is, there are these large language models like chat GPT that are commercially available. What you're going to do if you're going to build an application is, is kind of train that model on your use cases. But there are a lot of opportunities right now to apply NLP across your business, from chat related applications like automated phone calls or automated text messages, replies to emails, but also like less but also parsing documents. So any place where you get text based communication, right, I can read an email and understand what's happening. I can look at a pod and see an OS and D notification, and I can run, I can run automation off of that. So there are a ton of use cases, like across a business, that can solve this problem. So, like, you know, a good example is I had a customer. When I was a freight broker, they used to say, like, every every day they'd email me in the morning and say, like, are we good on our loads today? That's all I would say the emails, are we good on our loads today? And I'd be like, yeah, yeah, Willie, we're good on our loads. And so then I was like, Hey, man, I can give you, like, a login to the TMS, and, like, you can check all your loads. You can see who the carrier is, whatever. So I gave him this thing. I told him how to use it. I got an email every day, Hey, are we good on our loads today? And I was like, All right, man. Well, like, here I can automate this report. It'll email you a report that says this, that the other you know, and he would still email me every day, are we good on our loads? And so I started emailing him every day. Before he emailed me, I started actually just creating an email that would go out and said, Hey, we're gonna load today. Why? Well, because he not that he just wanted to hear me say, but he had trust in the fact that if there was any information that wasn't salient to him or that wasn't readily available to him, in a in an app, in an email, I would tell him, right? And so that's human to human communication. And then he also had the opportunity to ask me questions, because he's engaging me. So there are a number of opportunities there. The biggest challenge that companies run into is is in change management. And everybody hears about change management, they talk about it. It's really like, how are you going to how does your business? How does the business, part of your business need to change to get value out of these things. That's less a problem with things like natural language processing than other areas, other than other types of applications of technology, because it doesn't ask users to change their behavior, usually external users, especially external users like again, Willie didn't have to start logging into the TMS. He could just still keep emailing me, and I could say, Yeah, well, you know, these things are good. I could tell them load numbers. I could tell them, if you know who the carriers were, but you know, change management still is definitely something to consider, and how and in what areas do you want to refocus your internal users now that you've created these other efficiencies, and what is their incentivization like? So how are they not compensation? Compensation is a subset of incentivization, right? Natural, human incentivization. You've seen office space, yes, what's, you know, the line of he's like, I have three bosses, so, like, my only goal is to not get hassled if I make one mistake, three people talk to me about it. That's incentivization, his incentive, overriding incentive of everything else, even if the right decision would make the company more money or him more money, but it's the wrong process or whatever, he's going to do this thing because he's going to do whatever gets him not hassled, because it outweighs every other incentive. And so that's certainly a big part of thinking about how to apply things like what you're describing. So that was a long winded answer, though, no, it was great, because it's helping me sort of connect the dots. And I'm sure for a lot of folks out there that have heard, you know, a lot of these phrases, it's helping them, too. Now I'm curious, switching gears a little bit, you know, with your company and all the people that you're working with, what are some of the most, I guess, aha moments, or, like, exciting moments that you think is building for freight today and in the future? I think that what I really like that I'm seeing in technology today is like, there's a lot of disillusionment around technology that's available commercially right now. That's what I'm sort of sensing. And I'm actually kind of happy about that. And it's not the take of like, oh, you know, there's too much like of these. People in the industry who you know this venture money, and we need to clear out. The challenge is, like, there's a lot of most of the time, for what it's worth, like, when we go into a company and they're like, I hate my technology nine times out of 10, the reason they hate their technology is their fault, not the technology's fault, but And yet, like, that's act that's still meaningful, that still matters, right? They're not getting the value that they look for. But a lot of like, I think the trend in the last eight, nine years that I've seen is all around automation, and it's skipped a step, which is user enablement, right? So like, how do I make a user do their job better? And so there's been a lot of focus on automating things that aren't working the way that the underlying entity actually wants them to work, or needs them to work, to effectively grow or scale their business. And so I think that's something that's been really exciting for me, is folk people focus, refocusing on, how do I delight the human in the machine? Right? How do I create space? And I think that's actually going to make automation better in the future. Number one, because you're going to be able to automate things that are working. And number two, you have built more trust with your users, that their job can change over time, and that you can create space for them to do the things that humans can or should do, and that's not executed transaction, which we've sort of CO opted the term relationship to mean do a do a transaction, but actually a relationship like, talk about Blake Bortles on, you know, on, on the good place, or things like that. So that's something I'm really excited about. Is a trend in technology that, I think is that folks are starting to come around to and I think that, you know, with especially during this interview, you know you you are asking a lot of the deeper questions that I think helps companies save a lot of time and resources. I'm curious, though, for a lot of maybe the other players, or maybe some companies that are interested in working for or working with a company like yours, how do they know how to spot the bullshitters, like the bullshit tech, the bullshit consultants. How do you how do you sort through that to get to the good stuff? So my experience in this industry, in a lot in large swaths of the industry, has been that you have some really great business leaders that know how to build really great businesses, and they know how to operate really great businesses, but they don't understand their businesses in academic sense, like you have to sort of like and most consultants, most consultants take an overly a true, you know, like kind of true consultants, like management consultant, strategy consultants. They take, like, an consultants, they take, like, an overly academic approach, which is why people don't like consultants. They're like, you've never had to sit in our chair and make these decisions where, like, obviously, folks at metaphor, some folks at metaphor have, and so we understand it very pragmatically. But there's also, like, an academic understanding, like, too often companies are like, they'll, you know, they look at a technology partner or consultant, and they're like, hey, my My arm hurts, and the doctor will be, you know, and they and then, you know, do music like a doctor analogy. And it's like, All right, great. Well, here's some aspirin. Take this aspirin. Your arm will feel better when the truth is, like, they're having a heart attack. And they kind of like, if you understand the like, you have to understand how the body works, right. And but if you're just a person, and you go to the doctor, you're like, my arm burns, I expect that you are going to know. And so I think that the key really is, like, really, really drilling down to try and understand better their business. And ask, keep asking kind of questions. Keep peeling back the onion, because the most obvious answer, or reason that something is or isn't the way that it is is usually not the reason that it's that way. The pain is usually somewhere else. The challenge is usually somewhere else. And so I think that's, that's, that's certainly step one. What are the challenges that you're trying to solve. And then ask your ask your potential partners, really difficult questions about their experience, like what they've actually done, what types of problems they've solved, what impacts they've had, and and ask questions about what would make them disqualify a customer. I actually when we when we help customers select software, that's a that's one early question that will ask a potential vendor, hey, who's a wrong fit customer? And if their answer is a wrong fit customer is totally other industry, not super helpful, right? I want to know how what's a customer that kind of looks like me, but that's a bad fit for you. And that's true. I think of of consultants, you know, especially some of the like individual consultants, as much as it is technology vendors and whatnot. So I think that those are really kind of important things. Is really get smarter, on on, on how your business really operates in. More theoretical or academic sense, and Mary to Mary, with your with your pragmatic, really valuable operational experience, that's a pro, right there. You just brought it back full circle from the first question to the last or one of the last questions. Now we're here at manifested future of supply chain and logistics. So what's the what's your favorite thing that you've seen so far? So I'm not really a dog person, that being that, being that, being said, the puppy thing was cool. And I wanted to bring this up, since you had it on your LinkedIn, and you had the guy who runs it, yeah, you had Dan on an asset on the puppy thing, I think they should do like a puppy alumni event, right? So they should bring back the dogs. Like, bring back, are they now, like two years ago, dogs, so you could have, like a puppy pen dog. Pen, a dog pen. I just had some really great conversations. You know, it's, it's, it's, they've done a great job of bringing a lot of folks together, and so I don't know that anything I've seen is really cool, but I think the one thing that they do better than any other conference is giving a place and a voice for early stage companies. I have a real passion for that. Our customers are always kind of like interested in what is up and coming. They do an incredible job at that. So talking to some of these earlier stage companies that I that I either have talked to before or just got introduced to me, I think that's a lot of that's been a lot of fun. Ryan, it was awesome to finally get you on the show. This would be the first he's been invited several times. We just never formulated. It's been one of those like, we got to get you on. We got to get in there. Anyway, it's the truth. Duval, yes, till we die. Yeah, literally die, absolutely. Where can folks follow you? Follow you. Follow more of your work. Where's your favorite social media platform of choice? I'm not a big X person, although I am on x I underscore user prime. There's no longer a story than that one. But LinkedIn is a great place to find me. RYAN b Shriver, and you know, kind of never have state to reach out. I always love talking to meet new people. Heck yeah. Thank you, Ryan for coming on the show. Finally, thanks for answering the request. Welcome into another episode of everything is logistics, a podcast for the thinkers in freight. I am your host. Blythe brumlevery. We were proudly presented by SPI logistics, and we've got a great guest for you today. We have Garrett Allen. He is the co founder of load power load partner, and we're going to be talking about what else AI and freight it's the topic of conversation. It seems like you can't really escape it in the news or in your personal life or anywhere online. And so Garrett, you're the perfect person to talk to. Welcome into the show. Yeah, thanks for having me on. Absolutely not now we were just talking, you know, a little bit about your career backstory, but if you could just just give it, I guess the folks a little bit of a glimpse into, you know, how you've come into the freight industry, because you're not just this, you know, new AI company that's coming in as an outsider. You've been in the industry for a while? Yeah, yeah. So, I think I started in freight, how a lot of people do, which kind of fell into it coming out of college, basically started at a freight brokerage really early on. Was an early hire in Cincinnati, Ohio, is where they're located. I was like, the second IT guy, they hired there, there's like, 50 people. Long story short, you know, small brokerage, they started seeing a lot of success, and as they were trying to scale, they were having issues with their technology. So offered to, you know, hey, if you guys give me the right resources, we can build you a TMS and, like, help, you know, help this company scale. And they ended up betting on me. I built an engineering team. We built their TMS and, oh, wow, worked there for just under a decade, like, like, eight or nine years building that TMS. We launched it after a couple years of work, built the whole like, engineering team and left there. Kelly said, about eight or nine years and left. They actually just crossed over, like 500 employees and a billion dollars a year in revenue on the TMS we built. So learned a lot scaling there. You know, just how a brokerage grows and the problems you run into. And that was really great. And then from there, went and spent a few years at a digital freight brokerage and kind of the more of a tech focus side for a couple years. And learned a lot there. And, yeah, after that, moved on to what's now. Load partner. That's awesome. So you, you built a TMS, and you are still alive to tell the tale. How challenging was that entire process? How long did it take you? I imagine it took that's anything like they've got their companies I've worked for. It took a long time. Yeah, yeah. It is a, we always said that it's, you know, it's a living, breathing thing, like, once you even get past, like, beyond the hurdles of migrating from a legacy TMS, which was very old. They were on, for anyone that understands it's basically on QuickBooks, on premise and on Visual Basic, so very old TMS, and migrating, you know, their entire history of finances into a new finance system, moving all their TMS, everybody into a new TMS. It took us, I think, a little under two years, I think, in total, to get us moved on to the new system. I think probably close to about a year, year and a half, and then, yeah, after there's, like it, it never ended. You know, there's always a new feature, a new new customer that needs a new thing. It was always ongoing. Yeah.
Garrett Allen:Do you miss it at all? You know, honestly, there's, there's something to be said about, you know, being like on that front line. I think it's, it's really cool to be, especially at a company like that, seeing as much success as they did, and, like, growing so rapidly, to just have that, you know, it was like the typical startup life you hear about where, you know, it's like, Hey, we've got, we can make a whole bunch of money. Here's a great deal. We need to we need to be able to, like, do these things with our technology, you know, we need to support this kind of a customer portal, this tracking. And it was like, All right, you know, there's not a lot of overhead. It was really small team. All right, let's do it in like, two, three weeks. Let's throw it together and, like, it's really fun, you know, I think, you know, after time it gets it draw, it drains you. You You know, you can, like, keep that pace for so long, but it definitely was fun. So I guess, you know, speaking of, you know, sort of the digital front line, I
Unknown:not nearly as much as you have, but I have been diving into this world of AI for, you know, the better part of the last year, mostly on the marketing side of things, and it's helped me just so much in just sort of my day to day activities and how I think about business, it's also kind of hurt me in some areas, just because I feel like I'm almost like a little paralyzed to go after new projects as I'm like, wow, that's definitely a candidate to be replaced by AI. But I think that you know, which is talking to a lot of people, there's a ton of confusion around just what is AI. So from like, a very like basics, and I guess, you know, sort of for the audience listening, I want to frame this discussion in a few different ways. So first we're going to talk about the basics. Then we're going to get into a little bit of the use cases for today, for using AI, not only in freight, but maybe, you know, some use cases outside of it, and then we're going to get into a little bit of what we think, you know, the future is going to look like. But for folks listening, can just tell us what is AI, you know? What is it that phrase has been around for like, 70 years. How do you define AI? Yeah, so, I mean, AI, I think, could be broken down in a whole bunch of ways. The current, like wave of technology, is really around generative AI. Historically, when we've talked about AI, it's really encompassed sort of a whole lot of things, a lot of machine learning, where it's a lot more traditional coding of things that are a lot more you put in a and you get out b, and it's always that way, and combinations of that can make it sort of seem like intelligence, basically, right, like, think about like a video game AI. Then the kind of the new stuff is around generative AI. And what that is is it's essentially taking some concepts that have actually been around, but as we're applying to these large language models, like chat, GPT, we basically have this huge, like, tree of if then statements and all these weights. And what it does is it takes, like, given a set of data, it sort of tries to predict the next, following set of data that follows the trend. Like, that's the most simple way to break it down. So when we talk about, like, large language models with chat GBT, all it's really doing behind the scenes for chat GPT is it's getting a conversation between two people, and it's saying, Okay, now predict what, like user two would say, and then that's what chat GPT says. And so they frame it like, you know, it's talking to you, but in reality, it's just trying to predict the next word, basically. So boiled down, even with the AI video and image stuff, it's very much similar, where it's basically getting this, if you've heard of stable diffusion with image generation, you know, the way that works is basically it gives you an image with scattered pixels of like, just random. It looks like nothing, and asks the AI to just, okay, we expect it to look more like a dog, like, predict how those pixels would rearrange to look more like a dog. And it does that over and over and over again until it gives you your final result. So, yeah, basically boil down. It's really just predicting what it thinks the next word is going to thinks the next word is going to be. And there's just a lot of clever ways to use that, to frame it like, you know, that it can actually like, take actions and do things and seem like intelligence, basically. And that's that's a difference in how I was thinking about it, because, admittedly, I was thinking about it, okay, this is data based, and so it can't really have an imagination. It's based on the inputs that you're putting into the system. And you're telling me that that's, that's a little bit off base on on how it's actually processed, yeah, and it, and it actually, you know, yeah, I mean, it could definitely have sort of, like, an imagination. It's, it's hard to, like, put it, it's LMS are actually designed quite similar to, like neurons and like a brain where basically it goes to a point and it says, Okay, we're here. We know like XY data points based on the weights. Here, it's called weights. Basically like probability, we're going to expect, like the next thing to go down this path, right instead of the other one. And it basically just follows these paths until it gets to the end and gives you like, what the next letter is, or the next token. Um, basically, by taking, you know, tuning these models, it can take combinations of like, you know, paragraphs, thoughts that people have written down, images, whatever, and combine them and sort of create new things out of the input data that it's been trained on. Hmm. So depending on how you define, like, what a thought is, or what, you know, an imagination, is it sort of, can, I think in some ways, it's an interesting, like thing to think about. I think we really, really start getting abstract on, like, you know, talking about, does aI have thoughts? Or, like, you know, is it sentient? I think there's a lot of arguments both directions, honestly. So yeah, because there's a lot of people that say that they use like, Please and thank you when they're talking to chat. GPT, just in case, you know, the robot remembers in the future of who was nice to it and who wasn't. Is that a thing? Yeah, yeah. I mean, I don't think it's gonna actually remember who is, who is nice to it in the future, if it ever gets there, to be honest. But, yeah, it's funny. I think about, like, what the Google engineer from, what was that three or four years ago that was making the rounds about, you know, when Google was first crossing into this and their R and D signing, you're saying, you know, they've created a sentient AI, and it knows, remember? And it was like, making the rounds, like, this crazy engineer, oh my gosh. And, and then chat GPT came out, and it was like, Oh, wow, he saw this, you know, and you can see where he was coming from, when nobody else had seen this yet. So, yeah, it's, it's, I think it's super interesting, because for so long, especially in our industry logistics, it's been one of those things where a lot of the, you know, blue collar work has been theorized is that's going to be the first thing that's replaced with new technology, you know, warehouse robotics and autonomous trucks and things like that. But now, in such a short amount of time, it feels like the complete opposite, where the white collar workers are the ones that are probably going to be replaced sooner than anybody that's working in blue collar work. Do you see that as kind of the same thing? Yeah. I mean, definitely white collar jobs. Really digital jobs are, you know, ultimately, the things that most at risk, which really plays into mostly white collar jobs. But, you know, places, especially where we have text communications that have historically been sort of difficult. It's kind of gray area, right? We had natural language processing in the past, or we could sort of see what somebody was asking here, what they were trying to do, but it was really hard, like there was too much overhead. Didn't make sense to automate it. Just, just put a person in there. Now, with these large language models, it's so much more approachable. It's like we can just automate this. It's already digital. We already have the communications. The LM can understand this and say, what we're trying to do, it really is so much easier. And the other hard thing with with blue collar work is, you know, obviously, like, physical world, you know, going turning a wrench, picking a box up, you start getting into robotics. And one of the things with robotics is, you know, being able to, like, have a machine, balance something, and being able to be quick in response times and things like that. I think we're going to start seeing a transition into more of that stuff as some of these companies like grok, if you've heard of them, there's they're really making these LMS generate really fast, right? They can actually generate a response faster than like you or I could think about what the response is, which is completely different than the historical experience has been with chat GPT. Well, when we start getting into the scenario of an LM can now generate a thought like, you know, five times, 10 times faster than a human can. Well, now we can start applying that to robotics. Right now, a robot can actually play baseball or something, do something that requires more finesse, because it can generate thoughts at a speed quick enough to actually, like, do that. So I think, as we see like the generation speed for like tokens and these LMS, and not just LMS, but other AI kind of models increasing. We'll see more of the shift into some of that more blue collar work. And so you mentioned elements LMS a couple times, which I've been referring to them as, llms. And so that's, that's, you sound like that? That's, that should be the slang that I'm using. No, it's LLM, LLM large language model. Yeah, sorry, I'm kind of slurring that. But okay, I just wanted to. I was like, Oh, wow. So that's, I should be glad that I asked, No, you're good, because I was totally like, making a mental note, okay, don't call it llms anymore. Just call it LMS. No, no, I'm just, I'm just not pronouncing it all the way through, I guess. Okay, so let's speaking of those. You know, it feels like, you know, because, I guess, to sort of lay out the landscape, we have chatgpt, we have anthropics, Claude or not anthropics. But, you know, anthropic Claude, correct? But they just launched a new one. Then we have Google's Gemini, which used to be barred. And we have perplexity, and we have all of these different llms. Which ones are the ones that you're using the most today? Yeah, so that's a great question. So we actually load partner behind the scenes is sort of like a series of AIS. It's actually not just one. Some of the ones we use are, we actually use a lot of like Mistral models, if you're familiar with that, those are free and open source, so we actually don't host them ourselves. We pay for hosting, obviously, but they allow us to fine tune. We also use some of GPT. So we use GPT three, five, turbo and GPT four. And then we've been experimenting. With Claude three Opus. And actually the sonnet model is quite good. So we use this is kind of something not to completely derail, but basically we had load partner. We are a strong belief that, you know, a single AI model is not actually like the most effective way to give, like the best experience. And the best way to do it is actually to have, like multiple AI sort of working collaboration to give you a single AI experience where you have different like experts behind the scenes, sort of each kind of focusing on their own area of expertise and doing what they need, and then bubbling that back up as like a single response. It almost feels like that. This is for, I guess, millennials who grew up in a certain time, such as myself, you know, there was a big sort of console war, right? So it was Nintendo versus Xbox versus PlayStation, and it was really like the Battle of the exclusives. And that's who, you know, I would, you know, preach all day that that Sony has won this race and run won this war, is but in that, I guess, sort of competitive lens is that it kind of like a, maybe a good or bad comparison, compared to the llms that are going on today, that they have different exclusives, they have different expertise. Is that the way we should sort of be thinking about these things? I think it's kind of, it's almost like too early to tell and I say that because I think, you know, one of the big things with these different providers is that most of them are, are trending towards what open AI has already been doing, you know, especially around things like native function calling with new models that come out and, like, just the scheme of, like, how you interact with the model. Most, most of them have been just being like, okay, we're just gonna do a chat. GPT kind of does, because that's what, that's kind of the standard. Um, I think what we'll see long term is that we'll end up with something like what you're describing, where it's like, there's going to be models that are just better for certain tasks versus other ones, and that's going to be like, more of the separation, like the exclusivity, right? Like you're saying this one can generate better images. So if you want to do images, use this one right now. We're just like, not to the baseline yet. I think, like, everything is still like, advancing so rapidly. Something that could be like, not as good at images could be like the best at images tomorrow. And so it's like, too early to like, make that distinction, I think, but definitely in the future, the way I see it is that there will be a lot more models and kind of more what you're saying, Well, you'll have models that are actually better for certain tasks, and people will basically lean into those based on what they need. That's super interesting, because I heard from, you know, someone recently that they they pretty much said that we're going to reach a level where all of them are pretty much going to have the capabilities of a GPT four, right? Because this is what Google Gemini is chasing. This is what anthropic is chasing with their cloud model. I think it's Opus, is now what they're calling it. And so they're all going to reach the same level as chatgpt, and then we're all we're just going to reach parity. And then it's going to be a situation where you take your data and combine it with one of these models, and then you'll be able to work off of, I guess, that combined intelligence. But now what you're saying is that maybe you could still use your own data sets, but you're going to pick certain ones that are going to be better at certain tasks. Yeah, and think about it this way, you know, as far as when we reach parity, and, like, you know, like you mentioned, the Opus model cloud three Opus, and it's pretty good. It's, I think it's better than GPT four for my use cases. I've used it in but I think that goal post will just keep moving. Like, we'll never truly be at parity, because there's always going to be this one thinks a lot further ahead and can give you even more detailed explanations, but this one's faster, and this one's better at, you know, writing code for you. And that will always like, will never like, hit a point where it's like, Okay, it's good enough. It's gonna just always keep getting better. And so once we hit the point where the general, like, conversational aspect of it is, like, good enough. I think we'll start seeing those models become one more specialized, where you kind of get more of that exclusivity that you mentioned. And there's also different use cases too, right? So beyond just like the obvious, of like, this one's more detailed, or this one's better writing code, you also have other trade offs. Like, how fast does this one generate? Is it quicker? Is it slower? There's different use cases based on what your business is trying to do. About which one would you want? This one is writing emails. I don't care if it's slow, I just want it to write the best possible email. Versus this one's a chat bot. I want it to be fast and respond instantly. So I think a lot of those different aspects will ultimately distinguish them in the future. Yeah, it sounds like this is a good place to, I guess, to get into the, almost like the anxiety around some of these tools, you know, before we get into some of the use cases, I think there's, I can only speak for myself, and, you know, just the folks that I hear from. But it feels like there's either. Just a complete ignorance, like I don't want to know about it, or there's a fear there that this is going to replace my job. This is, you know, and in my own use cases, I'm seeing it as okay. There's going to be a lot of services that I offer now that's going to be replaced by AI. So how do I even plan for my business moving forward, if all of this is taking place right now, how do you sort of, I guess, that, you know, before we talk about load partner, because I imagine those that's going to come up in conversations where people are almost scared to adopt it, because it's going to replace them. Yeah, I mean, you know, AI really is kind of a new frontier, I think, a bit on technology. So I think with something so new, like, it's definitely understandable people be afraid of, you know, their job getting replaced. Or, like, what is really the implication of us using this? The way I see it is, you know, ultimately, it's gonna really help people do more with less. I think, you know, figuring out how you can basically use it to provide an even better service is, like, really the best way to approach it? I think approaching it with the intention of, like, how can I provide the same service for less or, like, put less effort into it is going to end up with, you know, people are going to do that, and it's going to it's going to happen. It's already happening. But it's obvious. Everybody knows. You know, if you go on LinkedIn, for example, and you look at through all those posts, you can immediately tell who clicked the rewrite this with AI button, right? People are always going to pay more and appreciate more like the like genuine service. I think that's always been kind of true, and it'll stay true so places where you can still provide, like, a really good service, but just like, improve it even more with AI. That's those people are going to be the most successful. And the people that are just trying to, you know, outright replace it, make it cheaper, you know, just pump out content, things like that. People are going to know it's going to be, you know, the cheap knockoff. Everybody's going to understand that, and they're going to basically be, they're going to be considered lower value. People are going to pay less, they're not going to pay as much attention to it. So, you know, I think looking at it that way, it whatever the business may be, I think it really kind of like helps go, Okay, how do I want to pull in? AI, it's like, okay, well, I probably don't want to just have this write my articles for me, but maybe I can use it to say, like, get pointers on an article I did write, and then maybe tweak some stuff from an article that I've already written. So you Oh, yeah, I didn't think about this little thing. Or, like, the way this is where it could be improved a little bit. Or, you know, others, there's, there's a lot of things around, like, taking quicker notes from a video, or, you know, getting transcriptions. There's a lot of advantages there. You don't nobody cares if you spent, you know, two hours getting the transcription written down for a video versus if the AI generated it for you, right? There's not really value there. So use AI, right? I think it's just weighing those back and forth as you're going through. Everything is just really important, yeah, transcripts have, you know, having the motto generated by AI, and just like meeting, note taking, apps have changed. Have been probably the largest impact on like, my career. Over the last year, year and a half, I remember having to just hand transcribe. And when I say hand transcribe, I would hit play on a video, type out what they said, pause, hit play on a video, type out what they said, pause, and it was just repeated over and over and over again. It took so much time, but now I can get that stuff out so much faster because of the, you know, these different transcription tools. So that is, you know, I guess a good segue into going to some of the use cases because you started building, or you've been building, a load partner, and kind of tell us about, you know, I guess, sort of the back story on that, what was the catalyst of starting upload partner and what it does? Yeah, so basically, with load partner, one of the big things for us is, you know, we feel like a lot of technology companies, especially in the freight tech space, are sort of trying to do too much. You know, there's a lot of apps out there. There's a lot of like, Hey, you got to download our app, or you need to use our tracking software, or whatever forcing sort of drivers, customers, whoever, to sort of come to them. And we've seen that there's a there's a real need for providing some more that service and automation through conventional methods of like SMS, phone calls, emails, you know, written communications. Drivers have smartphones in that right? So they can open a link on their on their phone. So basically, what we're doing load partners. We built a 24/7 AI load coordinator. And what it does is, if you want to start using load partner, you'd go through our onboarding process. You would fill out some things about like how you run your freight, when do you make check calls? How often do you want location updates from the driver, things like that. And then our load coordinator, with load partner, would, basically, based on those preferences, make calls out to the driver, send text messages. We have location links, we can send out. They can open to get a location and basically just help you manage, you know, your freight that's on the road. Um. Um, and the cool thing is, we're doing it all through, you know, SMS, you know, links to a website, emails. So we don't have, like, an app, they've got a download or new technology. The drivers got to understand it's all through, you know, coming to them. And so ultimately, we're just making communication between brokers and drivers, or whoever it's, managing freight a lot better and a lot simpler, and letting those teams focus on other things. And so for a lot of is it like a standalone app, or is it like an integration into their current platforms? What does it sort of look like from I guess, a user standpoint? Yeah, so actually, it's both of those. So we've built it so that if you want to use it standalone, we have a whole web UI. You can go in, you can go in, you can import your load directly through there. We have a number of import options for some popular TMSs as well as, you know, CSV, email. We actually can even take images, like if you have, like, a raycon or something, and import your information straight off of that. And then you can just run it all straight through load partner and get your text saying that something's going on or whatever. Or if you want to do integrations. We also have inbound and outbound APIs, so you can, you know, shoot us over your load information through an integration, we can send you the events back directly into your system. And in that case, we even have a drop in chat, so you can basically drop in a load partner chat directly into your TMS if you want, or just let your user pop it up on the side and basically talk directly to our AI and kind of get AI in your TMS for free. So, yeah, kind of either of those work. Oh, that's interesting, because it can just sit on top of because I imagine it would probably be such a, you know, a big hurdle to overcome if you were trying to convince somebody to move away from their existing like TMS in order to use your platform. But I think that sounds really cool that it actually sits separately from it, and you can get AI benefits without actually having to, you know, trust that your existing technology provider is going to create it the right way. Right? Yeah, like I said earlier, I already built a TMS once, and I'm not, I'm not trying to do that again. So please just, you know, integrate so that we can just use it that way. That's definitely the preference. And yeah, we can, I we've referred to it as sort of like a TMS light, because we have the basics in there. You know, you can manage a load appointment information the bare minimum you need, but we don't have anything around like billing. For example, you can't put billing information in the load partner and things like that. I was listening to for this interview. I was listening to your interview with on on PDQ America trucking for millennials podcast, which great episode, by the way, I'll link to it in the show notes. And they were talking about different, I guess, sort of etiquette around like check calls and how, you know, drivers will be pulled over for, you know, in the middle that, and then they get a phone call in the middle of the night asking them where they are, or they're getting these check call requests, you know, at seven in the morning, and it's bringing up, like all of these new questions that we've I don't think that we've ever really thought about asking around etiquette in this industry. And I thought that was a really great conversation that they were bringing up with you. So I guess sort of, how do you think about etiquette when you're building load partner in the features that are really beneficial to a lot of the users. Yeah. So it's actually a big one for us. You know, I kind of touched on it earlier about sort of coming to the drivers instead of forcing them into another app, right? It's just SMS, email, the usual. I think there's a lot of a lot of benefit there. And then we also have some stuff around, you know, driver preferences that we have planned for later this year. Not to get too far into it, because it is for later this year. But essentially, you know, we want to give a lot more control to the dry road. If we have, you know, load partner running your load, and it has its preferences, it knows where your location is, we can infer your ETA is based on certain things. You know, if you have, for example, Quiet Hours where we know you're going to be pulled over resting, we won't call you. We won't text you. You know, those are things we're going to surface to the driver to be able to set so that they won't get that midnight call, because we can see, hey, their last location was within an acceptable amount of time. Based on that, we know their eta is probably roughly right. We'll just call them in a couple hours when they wake up. And so, you know, again, we're not just helping whoever's like managing the freight necessarily. We're really trying to also give tools to the drivers to make it that, you know, they like running loads with load partner because it, it makes it nicer for them. They can, they can, equally, you know, text into their load partner number and immediately get responses about, you know, appointment time information, or pickup numbers, things like that. You know, load partners, load coordinators, available, 24/7 for them, just as much as for like a broker. Oh, interesting. And so who's doing? I guess the is anyone hitting send on these text messages? Is anyone, you know, picking up the phone and making these calls? Or is that all sort of automated through the software? Yeah. So it's dependent on your preferences. It can be fully automated. So we have, like, I said, SMS, and then we also have aI voice calls. So you know, load partner can actually, like, call a driver and ask, you know, what their eta is, or what their temperature is. If you want to make the call yourself, you can and then SMS. Same thing we have, like, the human in the loop of. Out settings so that, you know, a user can say, don't send anything to the driver unless I, like, review it and say, Okay, first same thing for other actions to make it that you can, like, kind of lock actions of the AI behind an approval process, sort of, if you aren't quite sure, because we know that, you know, jumping into full AI automation for none it can be a bit daunting. So, you know, trying to put the tools as much as we can into the user's hands. Yeah, for sure. And, you know, I've heard you talk about, sort of the, the analogy of, you know, for data management, of, like, garbage in, garbage out. How do you make sure that your data isn't garbage, though, yeah. So I have a lot of thoughts on this topic. The floor is yours? Yes, yeah. So, I mean, you know, honestly, one of the cool things with, you know, all these, all these models, is that they are pre trained. You know, if you go to chat GPT, and you go talk to chat GPT, you don't need to preface it by telling it about who you are and, like, what kind of stuff you want to talk about. You can just ask it a question, and it generates something back. They're all pre trained to a certain point. So for load partner, for example, our AIS, you know, we've built all these, like, freight tools under this freight platform, and then we kind of threw AI on it to connect the dots, right? That AI isn't just, you know, a chat GPT wrapper, where it's just, hey, chat GPT, can you tell us? Like, can you write a text message up for this driver? We have, like I mentioned earlier, a number of different models working in collaboration to give you that experience, and they're all trained already, not on your data, on, like, other data, like, how to run freight, so they understand, like, what's a load, what's a pickup, like, what's a reefer? Like, you know, it understands those concepts. So we don't need all your data to, like, train a model, like we've already done that part. All we need to know is, you know, hey, what's the load number? What's the driver's number? When are they picking up? When are they delivering, like, just those bare minimums that are, you know, pretty low hanging fruit for most organizations to provide. We don't need the last 10 years of your freight like, we just need to know what's on the road today. So, you know, a lot of these, you know, companies, I think, you know, data integrity is still important. Like every company should still, you know, keep that in mind. There's value there. But you know, to actually get started using AI, quality of your data is actually not nearly as important as with traditional machine learning. You know, back in the day, if you're building like, a machine learning model to, I don't know, calculate a rate. There's a lot of rate matching stuff with machine learning. That data has to be really cleaned up. Like, most of the work that goes into that is just building the pipeline to, you know, feed that data into the model and train it, and, like, have it understand your rates and predict stuff. You don't have to do any of that with all the new AI stuff. It's already trained. It knows what freight is and knows what to do. It just needs to know the details of what we're doing right now. So AI is actually a lot easier to do than it is like a traditional machine learning project, I would say. So anybody that's interested in getting into AI stuff, you know, I would say, definitely keep that in mind. If you've done ml stuff in the past and you're like, Wow, that was really hard. I don't really hard. I don't want to do that again. Or, like, that seems unapproachable to us. That doesn't necessarily rule out that it would be possible to do AI, because it's a lot easier. How, I guess this is going to sound like a dumb question, but how did you tell AI, like, how did you break it down from, I guess, from, like, a function standpoint, of, like, what you did, how did you tell it, what is a load? You know, what is freight like? What is logistics like? Is it as simple as just writing it out in a text document and teaching an LLM, so one of the cool things with AI, this is a great question, by the way. So one of the cool things with AI is it's very new. If there's, like, a lot of you know, try it out and see what works, kind of a an approach, but so that's one step, you know, like, what do you do? What do you try? Like, what do you do? Yeah, so, um, I've we, basically, we built, like, this AI framework that helps us to really quickly iterate on giving AI, sort of like tools and helping it understand, like, what it can and can't do, kind of bringing that down. It's, it's ultimately, at the end of the day, everything is a combination of fine tuning, which is where you can basically take a model, give it examples of like, here's what you got, here's what you said back, and you could make up when it said back, if you could just say, if this is what you should have said back, and you give it all these examples, and you fine tune it, and what that does, like I mentioned earlier, you know, these, these llms, are just these big trees of weights, and if you fine tune it, those weights actually get modified so that the results when you give it something more closely match to the data of examples you've given it. So we've done that in some cases where we've basically said, like, hey, if somebody says they want the latest location for a load, you should send a location link out to the driver, right? And we fine tune our models to, like, know all this. So then later, when it sees the the broker says, Hey, I need to, uh, where's the where's this load at? He goes, Oh, I know I was already fine tuned for this. Basically, I'll send that link out. And. So kind of that same approach with just freight and like, you know how what's a reefer? Mean, you know, what kind of trailers need? What kinds of things? What is a temperature? You don't need a temperature on certain trailer types, things like that. It's almost like, and I feel like I'm saying it's almost like, you know, drink every time I say that phrase. But it's gonna be, it's gonna happen a lot in this conversation. For a lot of marketers, I think that it's tough for them to get used to this industry, the slang, the terminology, but it kind of sounds like you've already programmed a lot of that slang and terminology into the machine, and so now it's already up to speed and up to date on it, where now it can see it can speak the lingo that everybody else is speaking. Yeah, yeah. That's, I mean, that's a big part of it. You know, with with communications like text, especially, people can be really short use a lot of slang, and so it's definitely important for a low partner to understand that and know what people are talking about. Basically, are you seeing, especially with the you're one of the first companies that I've seen, you know, deploy AI solutions in the freight world. Do you see any other companies that are doing AI right in this space? Are they existing companies, or are they new companies, or maybe a combination of the two? Yeah. I mean, it's hard to say, You know what is right? Like, kind of, you know it is, like the new frontier, like I said, Everybody's trying to figure it out. I think right now, which is, which is cool, I appreciate, especially, all the different approaches we are seeing. I would say the way we're approaching AI specifically, I have really not seen other companies doing it the way that we are doing it specifically. But there are definitely a lot of, a lot of people out there. I know parade does a lot of stuff around, like email parsing, for example, that I think that, you know, they're using generative AI in a way that seems, you know, hey, this is great. Bring users value. They're not trying to, you know, give them the world, which I think is really important when you're, you know, building with AI, you've got to be intentional about it and not just be like, Hey, this is AI. We'll drop it in, and the problem is solved. Um, so, yeah, I'd say that's, that's one of the biggest things with looking at, like, how people are using AIS, you know, what are they trying to actually solve? Do they need AI? Even within load partner, we have a lot of, you know, like I said, freight tooling we've built, that's not AI. It's just sending a text message, sending a location link, that stuff's not AI. It's tools our AI use, but, you know, it's us being intentional about the problem we're trying to solve. And so, yeah, I think that, looking at other companies, I can say it's hard to say, who's really doing it right? I think there's a couple out there there. They're making doing interesting things on it, though, for sure. And I definitely like watching all the different different attempts at plugging AI into existing processes. For sure, I love that you brought up parade because actually had their example in my show notes, because I saw a post from parade, because they have a new product called capacity co driver, and it's inside the McCloud TMS that marks. You know, I'm quoting their CEO here, but he said Mark's a pivotal moment in freight brokerage as 50 loads per day per person will quickly become the new normal. Now, when I, when I worked at a freight brokerage, 50 loads in a day like you were the rock star of the month, this sounds crazy that that would be the new norm for 50 loads per day per month, but if you're automating a lot of these different tasks, then it makes sense that that that person is going to be freed up to be able to do these other things. Is, is that a safe assumption? Yeah, definitely. Yeah. And like I said earlier, you know, I think that's a great example of, you know, if you can automate stuff that's like, hey, this, this is just, like, very transactional. We get this and we want to give this back to them. And, like, there's not a plus one service to offer here. It's just get this done. You know, that's a great example of, hey, now we can do 50 of those. And, yeah, well, there's this other in as you were just talking, it brought up this other point that I heard someone make that if, and just theoretically, they're saying if we're going to be freed up. If AI is going to help us free up this time from these mundane tasks, maybe those mundane tasks are important because it allows us the more bandwidth to handle the larger I guess creative tasks better, because now if we have more time to be more creative, then that would lead to quicker burnout is kind of what this creator was making. The argument is that I can't think like that all the time, like sometimes the mundane task helped me to avoid the big thinking, and then I could get back to it later. Do you think there's an argument to be said that that it, you know, it almost might lead, you know, to, I guess, burnout much quicker if we're just focusing on these bigger tasks all the time. Maybe it's definitely an interesting thought. I think what we'll end up seeing, if I just, if I had to guess, is just a lot of those mundane tasks will get automated through AI, but some of the more complicated tasks, like, you know, these crew. Creative ones that are like, Hey, this is a I got to spend a day really thinking how to do this. They become more mundane because we have better tools for them. So like, even though we have to now, like, you know, hey, AI can't solve this all the way it could still maybe solve it 80% and you're just doing that last 20% and so stuff that you know, just because AI can't do it doesn't mean that it's necessarily going to be a really hard task. It might be that, just like that final bit needs to get, you know, finessed by a person actually looking at the response or, you know, filling out the final details. So I think there's still going to be, you know, mundane work to do, even with AI around, and that goal post will just continue to move. You know, the tools will get better. Will solve harder problems. The harder, problems will get easier and like the cycle will repeat. I think if someone is listening to this interview and they're thinking about, Oh, would it load partner? Sounds like a good solution for me, how should they start preparing their company, their business, maybe even just sort of the mindset within the company, how should they start preparing themselves internally to be able to start hiring external sources like yourself. Yeah. So, you know, good APIs is definitely a big one. You know, understanding sort of like how you get data in and out of your system again, you know, we don't need you don't necessarily need the whole world of like, your 10 years of history, but just understanding how an integration like that would work. The other thing is, you know, load partner is really like I kind of explained earlier. We take your preferences on how you like to run freight, and then we basically try to mimic that within load partner and run it the same way. So understanding what that looks like for your company well is also important. If you're like, we don't really know when we do check calls or why, then it's gonna, we're gonna have a hard time replicating that. And then, yeah, another thing I kind of touched on was just, you know, being intentional about the problem you're trying to solve. You know, if, if you're want to bring AI in load partner or otherwise, you know, really think about, like, what problem you're solving. If you're just pulling in AI to pull in AI, you're probably not going to get a lot of value out of it. It's really good to kind of outline like, what you're trying to solve specifically. You know, hey, we, we need to scale up our our load count per rep or whatever. And we, we don't have like enough to like whatever we need to bring in, basically, AI to, like, assist this person who's going to have a lot of loads this month. Okay, we can pull load partner in. But if you're just like, hey, it would be fun to have a chat bot, they can answer questions about our knowledge base. It's like, well, like, do you really have an issue there that you're going to solve with a chat bot? Right? So it's just, it's really important to think about, like, the specific problems you're trying to solve with AI if you're going to bring with AI if you're going to bring it in, because there are scenarios where, again, back to low partners. You know, some of our stuff are location links and sending text is traditional programming. That's not AI. And there's potential that even non AI solutions will solve the problem that you have. So, yeah, it's almost like a good process audit is in order, for a lot of companies, in order to figure out that maybe, is that a good starting place for them is just audit your processes. Do you even have them documented, if not document them and then see where AI plays a role? Yeah? Yeah. I mean, really, you know, the way I see it is, AI can is going to be able to like, fill in for like, if you're going to onboard a new person and to your team. You know, how would you explain to them, like, how you want to run a load? If you can't do that, then you also can't explain that to a system that is going to try to replicate it. Right? Same thing with a lot of AI. Most of the AI solutions need a similar like, guidance, and so if you can't explain what you're trying to do, basically, the AI can't magically, like, make the decision of what you're trying to do for you, what does sort of the pricing model look like for load partner? Is it per user, per i guess, token, or maybe a combination of the two? Yeah, it's a good question. We're still working with our early design partners to sort of like finalize that, but mostly the way we look at it is per load. I think that's how a lot of freight brokerages run their costs. Is really cost per load. So we have a couple different features that that may be priced differently based on the needs that we have. Like I mentioned, we have aI voice calls. If you don't need AI voice calls, that changes the pricing for us too. So there's, there's a lot to work out there, basically, but cost per load is definitely how we're approaching it. Interesting, and you're still on a wait list, correct? So you talked about your design partners, and those are the companies that you're working with in order, I guess, maybe to come out of beta. What later this year? Or what does that time frame look like? If you have that in mind? Yeah, yeah. So we still have a wait list. Right now. We're planning to come we're definitely going to be out of beta. We'll be open this year, for sure. I would expect sometime in q2 that we would have, like, a sign up available, or if you were interested, you can sign up and just use it on your own. Like I mentioned, you can use it outside of your system. So what we're really going for is somebody can go up, sign up for load partner, and literally, just start using it without even, like, having to go through. A sales cycle or anything. Just go sign up, put your information in, get a subscription, and then just start using it. We'll have inbound and outbound APIs that are completely self serve, if you want. So we're shooting for that later this year. I would say sometime in q2 the gates will be open a little bit better. So, oh, that's cool. Yeah, that's exciting to watch. And I love that model of just letting people try it and give it a test drive before having to talk to anyone. I know that for a lot of, especially folks that are going to adopt, or more willing to adopt this kind of technology, they maybe don't want to hop on a sales call. They'd rather just give it a test drive themselves. And I'm sure maybe you're seeing a lot of that same thing. Yeah, absolutely, yeah. We, we have a lot of confidence in the stuff we're building, so we definitely want to, you know, just get it out there and just get it out there and have people experience sort of that, like, magical, like, Wow. This will just, you know, see that driver's late and, like, send me a text and like, you know, see that in action. We definitely want to just, like, give that experience to people so they can understand So, and what is your your team structure look like? So your co founder, so I imagine that there's another founder that you're working with, it is, are you, you know, they have a team, maybe offshore or internally. What does that look like for you? Yeah. So right now, we are pretty small team. It's me and my co founder working on this, and then we have someone helping with marketing, and then someone helping with, like, customer support, basically, you know, helping like the the on call kind of things come up. Wait, so how are you handling your customer service in marketing with AI, I'm curious so cus So customer service is, you know, when even with, you know, AI, stuff comes up. So we still have, like, a customer service to help with if somebody has questions about how to use the system or something's not working correctly. And then with marketing, uh, yeah, we actually have, uh, we have someone actually, you might know little Miami marketing, Chase Osborne, if you're Yeah, he's been helping us, so he's doing a fantastic job. So yeah, all of our posts on Twitter and our funny memes, that's all, it's all him. So that's, oh, that's great, because it is great marketing, and that was one of the big reasons why I wanted to have you on, because it's called load partner, and we kind of joked around before we started hitting record that you kind of hit on the, I guess, the capitalization of the cowboy market, because Beyonce is new country song and all that. So it's a really cute branding. And I love how he really dives into our braces, the meme culture that has taken over freight, yeah, yeah, it's so good. It's, it's, we've all enjoyed it, so it's been a kick for us, too. So that's awesome. Shout out to shout out to chase. Good job, because the marketing has definitely been noticeable. So I know that's probably, hopefully that that's a good compliment to him. It definitely is, at least from my side of things. Now let's switch gears a little bit and talk about the future, because I want to know what is all hype in AI and what do you think could be around for the long haul? Are we going to be still be using llms in a few years, or is that really difficult to tell? That's a good question. You know, I don't know. We'll be using some form of something like an LLM, probably, I think there could be like levels on top of that, where, you know kind of what I was saying, where we have like multiple AIS working collaboratively to, like, give you the one AI experience. I think some kind of architecture around that might become more standard. So it's not just one. LLM, it's like mini somehow. I do think that, like I mentioned earlier, the generation speeds with companies like grok are gonna, like, really make a difference. Because, you know, right now, you know, you ask chatgpt something, you have to sit there and watch it, like, type its response out basically, right? It's pretty slow with grok, we're kind of crossing into that, you know, an AI can think faster than a person can think, and that just really opens up a lot of opportunities for real time applications like robotics or even just better chat experiences. When you can ask an AI a question and it can, you know, go search five websites, do all this research and give you a response back faster than you can even, like, think about what you what you asked it, that's gonna be crazy, right? Like, that's a whole nother world. So I think we'll, as we get closer and closer to that, the AI is just gonna become more and more, you know, it's just gonna be, like, mind blowing. I think, I think we basically haven't even seen the beginnings of, like, how crazy the technology can really get. And when you're talking about grok, you're not talking about, like, Twitter's Grog, you're talking about the other grok, yeah, yeah. I should have clarified. It's a good point, yeah. So there's a company called grok. It's with a Q, G, R, O, Q, and essentially the short is, they are, I guess, I don't know what. I guess the right way to put it is, basically, they have their own chips that they manufacture, or they are designed, I guess, that are specifically designed. So if you're familiar with, like, a computer, you've got, like your processor, and then you have like a graphics card, right? And they're specialized at graphics, is for, obviously, your graphics. Well, they've created language processing units, which basically are built to run llms And so. So essentially, they can run, they can generate responses from llms, like, you know, 10 times faster than the competition. So I think the numbers are, don't quote me on this, but I think it's like, chat GBT is like 40 tokens per second. So a token is like roughly three letters, and then grok with Mistral just hit, I think, or what I think it was, Gemma, maybe, or mistral. They, like, just hit, like, 400 or 500 tokens a second. So they you can, you know, ask it to, like, write you a story. And, you know, with chat, GBT, you see, like, right in the paragraphs, with grok, it's like an H, like a web page loads. It's just like, there it is, the whole thing, uh, which is crazy, because then if you say, Hey, you build something like a React model, or something where you have, like an AI that's actually like reasoning and like acting and reasoning and like looping on itself, well now you can ask it to do something and just like that, boom, it can have done 10 things instantly, which is crazy. So why aren't, you, know, like a chat GPT? Why isn't it using the chips specifically designed for llms. I think there's just new so I know. So I know previously they were using chips from Nvidia, which is why Nvidia, see no stock is crazy right now, because all the AI stuff, I have a feeling they have something probably up their sleeve. They haven't mentioned a partnership with grok yet. I know that grog has been like, prodding at them on social media, just been fun. But, yeah, I don't know. I'm sure that they have something that they're working on, because it's been, you know, making so many waves, and it's really cool. So I'm sure something's coming. I think it's just a matter of, again, this is such a new frontier, and there's so much new here. It just, they just haven't done it yet. Basically, it's just brand new. So we've talked about, you know, we've covered a lot of ground so far. It's been on the the chip side of things. It's been on the large language model side of things. But then there's also, like, the open versus closed side of things. Do you see enterprises building their own groks internally, or their own chatgpt internally. Or will they really use some of these tools in the best use case scenario, like what we were talking about earlier with, where maybe one is better for images and one is better for documents? Is that the kind of the, I guess, a hybrid future that you see, I think it's kind of hard to tell. I do think that, you know, we're going to see a lot of AI providers pop up that are specialized in industries, you know, like load partner, for example, that we're going to sort of this AI provider that specializes in, like, running freight on the road. Think there's going to be a lot more of those, just in different industries. And companies are going to kind of like, move towards like, hey, let's just find a partner that really is keeping up with AI. It's really fast moving. Let's just pull in their systems and not build it ourselves. But I definitely think there will be companies that run their own. I think it'll be a combination of using general purpose models like chat GPT to just solve like a simple use case, and then you'll have more advanced ones, where people are these companies are actually trying to build their own internally and train a model from scratch to do some, you know, specific use case. So I think we'll see a little bit of everything. But I think the most popular will be, you know, finding a good AI partner for your business. It will be like the thing that makes the most sense, or like a internal AI ops team is, is kind of, for other companies that have come to me and asked me like, well, you know, how do we even start implementing this? And I was like, well, just start an internal assign either one person as an AI ops manager to figure out the different use cases and stay on top of the news, or maybe it's an internal team, you know, the subject matter experts from each department that are getting together regularly and convening on how they can start utilizing these tools. Do you think that that's a good pathway for businesses to use as as they think about AI adoption? Yeah, definitely. You know, if, especially like a medium, larger business, it makes a lot of sense to have, like, an internal to team that's, you know, tasked with this. You know, speaking strictly from the engineering side, we have a lot of the similar things right, like a data analytics team or a DevOps team that does, like deployments to production. Having something for AI is it makes sense. You know, it's a really fast moving field. It's hard to keep up with. And there was a quote of open AI founder, or one of the founders, Sam Altman, and he said about 95% of marketing tasks are going to be handled by AI, which to marketer and freight, you know, it kind of freaks me out, but it's I try to avoid, like, sort of the doomerism around it, because I think, to, you know, my own examples, like, you know, social media, for example, social media managers didn't exist 15 years ago, that was a job that evolved from tech innovation and tech evolution, and so I guess, how are you combating, maybe the doomerism that exists when you're talking to customers, maybe fear of being replaced within your own organization, or maybe you're building a business that might be obsolete in five. Years. I think that's the questions that a lot of folks who are studying this are having. So how are you, I guess, sort of coming to grips with a lot of those questions, or how are you thinking about it? Yeah, no, it's good. I think, kind of, like what I was saying earlier. I think, you know, even, like, with marketing, right in the 95% I don't, I don't know anything about, like, where those numbers come from, but just thinking about it, you know, you could go on today and chat GPT and say, like, write me an article. And like, generate me an image for the thumbnail. And like, if you posted that article in that thumbnail, people would immediately know you generated this with AI. It's not, this is it's not genuine. It's kind of like the same thing I've read eight times with different like, things plugged in. So I think you know that same thing applies everywhere, you know, if there's, if there is a, you know, a service that's really transactional, where it's like, hey, we just do, we get a, we give B, there's no like thing we can do better here. They just want this done. They don't care. AI is great. If you're like, hey, we're trying to make a sale. We're trying to land a customer, trying to give, like, a really excellent experience. Just plugging in AI is probably not the answer. Maybe it can help, but just plugging it in isn't really the answer. You want to use it to give a better experience, but not replace it. So I think when you're looking at AI again, it's just being intentional about the problem you're trying to solve, basically, and not just trying to make it easier for yourself. Because people can tell and they and the thing with people is they've always, you know, appreciated that the genuine person, the real original content, stuff like that. You can always tell, you know, even, like going on YouTube videos and stuff like that, and you've got how many channels on YouTube that are the same exact channel with, like, a different person that's doing the same things, and it's like, they all get lost, because it's like, it's the same thing, the ones that are popular, the ones doing it different. And I think that's just always going to be true. Yeah, I would agree with that. And another, I guess, sort of use case that I saw that blew my mind, and I don't, I don't think this is exactly true yet, but it involves a, you know, the conversation around AGI and that, I guess, more expanded large language model of currently what we're using, and it was basically like a lot of pixel characters that were sitting in a pixelated office, and it looked like a little video game. And you had different departments, you had a marketing department, you had a sales department, and they're all these little characters that are all working at their individual desk, and they're all talking to each other independently and solving problems. Do you think that that's a real sort of like, Would I be able to build my own sort of mini marketing agency where one team is handling SEO, one team is handling web design? Is that a future that that's in our near is that a near future? I guess it's definitely a future. Is it a near future? I think it's hard to tell the tech is moving so fast. Possibly, I think you're still going to run into a lot of the same issues, where, if you've got just an AI handling SEO, for example, like it might not do the best job it's going to do, like, the bare minimum standard kind of, if you really want to, like, crush it, you probably have to, like, get a person in there. But I do think a more near future is that, you know, I kind of mentioned again with Lloyd partner and having, like, the different AIS work in collaboration. It's, it's really approachable to have, like, an AI that specializes in, like, SEO, like just, it just does SEO. I think a more near future would be a company that has AI sort of broken out similar to, like, whatever departments they have in place, or, you know, like, how you do your marketing agency where you have, like, Okay, I'm gonna be doing SEO stuff today. I'm gonna go, like, work with my AI that focuses on that specifically to, like, get feedback on it. And, you know, a company might have an AI that's trained for helping with marketing at different parts of marketing, and that's what those employees use to, like, help them out. Um, but I think having, like, a full blown company that's like, entirely AI run, essentially, is probably not quite as near future, just because, um, yeah, there's just too many edge cases, too many things to think about. I think we could probably, you could probably, like, technically, do it, it would just not be, it would just be pretty poor. Basically, yeah, that makes sense that that's probably the images that I was seeing. But are they? What is the actual, like, work output, and is it decent enough that somebody would actually pay for it? My guess right now would be, no, it would not be something that that people would pay for it. As we were talking about Google search, you know, I thinking about Google and, you know, the sort of their big snafu that they had a couple weeks ago when their launch of Gemini, and, you know, all of the, I guess, the the PR backlash of their programming, I guess, where do you sort of see Google's standpoint, or alphabet, I guess, as the their parent company. Where do you see them fitting into this AI arms race? Do you see them being competitive, or was that snafu that they had a few weeks back? Is that sort of indicative of just, I guess, sort of larger data set problem? Yeah, I don't know. I've actually, you know, to be honest, I've been really surprised with how far behind I've. Like, they've been since sort of day one. You know, Google's always been, sort of, like the AI, like forward software side, especially company I was really shocked at, like, how poor their first like reveals were, and like how they've just really struggled, like, the whole time kind of here, you know, I think, honestly, that they, I think they are just legitimately behind at this point and just try and catch up. And it's just so everything's moving so fast. It's just, once you're behind, it's so difficult to catch all the way back up. So, yeah, I don't know. I think, you know, one of the things that they probably do have an edge on is they're talking about some of these smaller models, and like, getting them into their phones and like, kind of going to groks, you know, chipsets that are made for llms, something similar for the phones where they've got these AI processors, and the phones that help, you know, run these small models directly on the device quickly. They probably have an edge there. So maybe not so much in like the great, general purpose everything models, or even, like, you know, your enterprise stuff, but more of like the small, fast can answer a quick question, like your Google Assistant kind of stuff. I think that's where they might have something, you know, sooner than later, probably, which is crazy, because they have access to so much data. So it boggles my mind how they could be so far behind on this. It just feels like they they've kind of, you know, just got caught with their, you know, their pants down, essentially, and just caught, caught surprised. Or maybe they just don't want to mess up their entire business model, which all comes from search. I think it's something like 80% of their revenue comes from search, which is crazy. So they probably don't want to upend that so, but then they're going to get replaced by it anyway. So it's, it's really weird to watch them struggle over, over these last few months. Do you think that they recover? Um, yeah, I think that they I think they will, just because I think, like, the the Android devices just give them such an edge on, like, getting an AI of any kind into someone's hands. You know, chat, GPT and like Claude three. Like, benchmarks wise, they look great. Enterprise wise, they look great. Nobody's really done like, a a Google Assistant, a Siri, an Alexa, yet that's like, really generative AI, like, it's still nobody's quite done it. And Google has, like, an experimental one out that I've messed with a little bit on my phone where it can actually replace your Google Assistant with, like Gemini. So it's actually like generative AI, but it doesn't have all the tooling hooked up so it can answer questions like chatgpt, but if I say, like, what's on my calendar today? Can't tell you yet, but it's like coming soon. So if they can get like that, they can bridge that gap before anybody else. I think that will get them kind of caught back up, in a sense, even though it's sort of a different area, it'll just be like, okay, Google's doing something with AI. They were the first to like the personal AI assistant, sort of, so that's what I would expect. I know there's some like, rumors about Apple doing AI stuff behind the scenes and doing different stuff like that. So I did, is going to be a lot of interesting stuff on that, like personal assistant, AI stuff within the next, like two years, probably. How do you keep up to date with all of the the news that drops and experimenting and, you know, trying to focus on building a business, but also trying to stay up to date with all of these different tools? How are you managing it all? It's hard, you know, honestly, sometimes it's a lot. I think the big thing for me is I just, I follow a lot of the companies on, like LinkedIn and Twitter to see when they make announcements. Hacker News is always a go to for me to see, like, kind of the new tech news for the day. And then otherwise, yeah, I mean, there's stuff like, you know, grok when it first came out with the fast token, stuff like I just saw by chance, somebody sharing that on Twitter one day. And I was like, Oh, what's that? And then like, the next day, or whatever, they announced, like, really fast with the Gemma model with Google. And I was like, Oh my gosh, this is crazy. And, yeah, it's just, I guess it's just kind of being, like, in the news feeds a bit, and, like, just trying to keep up with, like, the AI tags and trying to weed out there's, you know, there's some people I feel like you can tell, like, post more regular stuff that like is actually news versus, like, some people that will post stuff, and it's like, this was from like, a week or two ago, you know. So like, the AI newsletter roundups, like, I feel like I'm subscribed to, you know, a half a dozen of them right now, and they're all reporting on the same thing. So probably time to go in and clean up my subscriptions a little bit. I am curious, you know, a couple last questions here, what tools, I guess, in the AI realm, are there any AI tools that you're using outside of load partner? You know, honestly, funny enough, I've actually done some transcription stuff before, like last year a little bit more Reed was doing, if please advise and like the Read, read and all them, they we have a tech for a Tuesday thing they do on the discord. And last year, for a month or so, we were doing like sum those nights. And so it was really cool. We were basically using Amazon to transcribe it, and then we were actually feeding the whole thing. And the Claude, my anthropics Claude to get a bullet pointed summary. And I was really impressed by that, because we would have, you know, three, four hour conversations, and we would just plug it in, and it would give us, like, here's like, the 10 topics you covered, and like, what people said sounds like, Oh my gosh. So that's cool. And then, yeah, I mean, I use GitHub copilot for programming. You know, it's, it's funny because, you know, I would have probably said, like, there's no way I would ever be that guy that uses that but, oh my gosh, it's like, life changing. It's so easy. How so, what does it do for folks who may not know? Yeah, so get up. Copilot is basically like an LLM that helps you write code. So what you can do is, like, if you have a file open, you can just literally add a comment and be like, I want a function that does this, and it'll spit out a function that does that. And it's not perfect, but it does a really good job, especially if you're like, I need to write a function that, like, I know I've written six times and like, I just, I still want to type it. Obviously, you just write the obviously, just write the comment, let it do it. And it's funny. One of the things I was telling, I tell my co founder is, you know, in programming, there's a lot of, you know, back and forth about how much you should leave comments, and like, what does or doesn't need comments? Well, you know, five years from now, I think it's going to be funny. We go back and look at this old code that people have written like myself, and you're going to see comments that are actually like, prompting AIs. And it's going to be like, you know, a comment somebody left behind that was like, write me a function that says, blah, blah, and then, like, there's a function like, oh, like, he was using an earlier version of co pilot, probably when he wrote this, because that's clearly like, an AI prompt. But yeah, it's funny. I love it though. So, you know, it's helpful. No, it definitely sounds super cool, especially with your expertise and your history within the freight industry. I think you're, you know, you're coming from a perspective of, you've already built it manually, so now how, if you were to build, I guess, sort of a modern day TMS, like, what would that look like? And it sounds, you know, a lot like, what load partner is a, you know, a perfect complement into the day to day life of, you know, brokers and carriers out there. Is there anything that you feel is important to mention that we haven't already talked about? Oh, no, I think, you know, just the biggest thing is you're with AI, I think just be intentional about how you want to use it. And, you know, I think find a good partner, and that's just really important, as far as, like, you know, staying ahead of sort of the AI hype training can be really easy to get sucked up into and be like, we got to plug AI in everywhere. I think it's just, you know, think about what you're trying to solve and, you know, find a good partner that knows what they're doing to help you solve that problem with AI or not. Yeah. Well said, where can folks follow you? Follow more of your work. Sign up for load partner, maybe. Yeah, yeah. So you can find us at load partner.io just one word. You can email me, always happy to talk about this stuff. Garrett@loadpartner.io and you can also find me on LinkedIn and then also on Twitter. Garrett underscore makes awesome. We will put all of that in the show notes, just to make it easy for folks. But Garrett, this was an awesome discussion. Thank you for entertaining my super basic intro level questions. But I think hopefully this was a good educational lesson for the folks who have been curious about AI and freight and maybe want to learn it a little bit more and be prepared for the future. So thank you for your expertise and your time. Thanks for having me on. It's fun.
Blythe Brumleve:Welcome into another episode of everything is logistics, a podcast for the thinkers in freight. We are proudly presented by SPI logistics, and I am your host, Blythe Brumleve, today we've got a great discussion to have. It's all centered around visibility, which I think is the buzzword that you hear at every conference. But we are going to be talking about, and I think you guys coined this, the container operating system within global trade. We are talking to Gnosis freight. CTO. Jake Hoffman, so Jake, I know we've been trying to make this happen for a while. So glad to finally have you. Yeah. Blythe, happy to be here, and everything you said is totally correct, and can't wait to dive more into it. Awesome. Well, well, first, before we get into, like, the serious side of things, you are based in Charleston, South Carolina. I have a very guilty pleasure of watching the show southern charm. And I have to know, do you watch the show? Do you see any of these folks around town being a hot mess? Yeah. So my wife is my wife, Aubrey is a huge Bravo person, and she watches like, you know, the real housewives and all these and so southern charm is one of the ones that I do watch, because they go to the same restaurants and the bars and all this stuff that we do. And so it's, I mean, I watched that with her, and then it's, I mean, Charleston's so small where, if you go to King Street, like right downtown, then you go to any of those restaurants or anything, it's pretty likely at some point that you run into some of the people from the show. It's pretty funny. Are they? Are they as of a mess on a regular basis as they are on the show?
Jake Hoffman:Maybe I don't see them being too much of a mess. I think they've they've matured. And are they have their public persona done pretty well. They're all super nice, though, so I think met them all, and they're all great to meet and super nice in person, which is very referred.
Unknown:Watching. Okay, last southern charm question, because I'm sure people listening are probably going to be annoyed, but who's your favorite character on the show? I have a lot of respect for Craig. I think Craig is super nice. I've met him in person in Charleston a few times. He's super nice. And, you know, he's he's on the a couple of the other shows that my wife watches, and all those things, but he's, like I said, all of them are nice, but Craig is the most like anytime I've met him in person, he's friends with some of my mutual friends in Charleston, everything. He's never stuck up or arrogant or anything about ever whatsoever. That's super cool because, and that's cool to hear too, because he's definitely had the most, you know, come up story for folks who I'm not going to explain history actually, let's move on to the, you know, the topic that people probably clicked play on on this episode for and, you know, I hear this phrase. I've given a, you know, a moderated a couple panels around visibility, and I hear the phrase thrown around a lot. But can you define visibility for us in the freight world? Sure. Yeah. So I think Gnosis as a whole. You know the you can lump us into the category of visibility, and visibility means a whole lot of different things to a lot of different people. For us, we focus on international we focus on the international journey of a container. So from the time a container is loaded at a factory, either overseas or here, if it's an export, going somewhere else, to the time that containers empty returned. And so visibility can be the the milestones of it gets loaded, it gets, you know, truck takes it to a port or a terminal. It gets loaded on a vessel, and then it's in the middle the ocean. So the visibility would just be the hey, I want to know the milestones when they happen. And then what's my eta for when it when it's going to get to its destination. And so that's, you know, visibility as a whole. We drill a little bit further down into that, into, you know, you mentioned container operating system, our CRO Michael coined the term container life cycle management, is what, what he calls it. And so now we've, it's kind of a, he says, like we invented our own new category, and now it's kind of caught on. And we, we have really doubled down on that. And we think that, you know, visibility is important and getting the data around where your shipments are, but what's even more important is the execution piece beyond that, right? So visibility helps you understand what's happening, and execution helps you actually do something about that. That is a perfect sound bite. I'm just going to go ahead and thank the post production process for later on, because that is going to be the intro clip that we use for this episode, because it's always bothered me or not, maybe not bothered me, is the right phrase. But curiosity. When I check in Amazon, and I can see the truck that's delivering my product is six blocks away, and there are four stops for me, and I can see that level of visibility down to the granule, granule level of when a package is going to be dropped off at my porch. However, that doesn't seem to really exist. Or maybe I'm wrong, that that doesn't seem to really exist in container shipping. Is that accurate? It is accurate, and it's that's accurate to say, and it's the reason it doesn't is because it's so difficult to achieve that. And the reason it's so difficult is because you think, like Amazon for the most part, or the USPS or UPS or FedEx or someone, all those companies are so big, and the parcel network of you getting a package delivered to your house is those things are so small that they can control the entire infrastructure from end to end. So like FedEx has an actual truck that's theirs, that's coming to your house and making a delivery, right? And so if they have the plane that has the FedEx logo on the side, and they have the truck, then they have all their own assets and their own infrastructure to handle all this. But when you get into international freight, you see the giant ships that are in the ocean and in the harbor. You see all the trucks that are in and out of ports and picking up containers and doing all these things. The infrastructure that's required to do that is so large that there's no way for one company to do it all. So you mean freight forwarders are like the best example of someone who attempts to do this. And they do it through contracting all the different players. They contract the ocean carrier, they contract to drayage, the truck that picks up a container, and then they even contract warehouses and do those things. But there's still all these actual individual asset owners that all have to work together to make it come to fruition. So the only way to get that end to end visibility that you know your Amazon experience for international freight, is to tap into all of those individual sources and get the data from all those different places and put it in one kind of seamless data model that you can show to the end customer, which it sounds like, I mean, in theory, it sounds like it should be just as simple as taking a little apple air tag or something very similar, just throwing it into into a container. Why isn't it that easy and then sharing that data with all parties involved, right? And that's some people are getting better and better at it. You know that some of the ocean carriers have announced that they're going to, like Hape Lloyd, announced they're equipping every single container with IoT devices. I know that CMA has some mares custom that they do for reefer specifically, and their plan is to roll it out to all containers. But. Even if you get to where each container has, like, a GPS tag on it or something, we can kind of get there now, at least when it's on the water and when it's in the terminal and things through, like AIS satellite data telling you where the vessel is in the ocean. You know, you open up maps and you can see where it is on a map. But if, even if you do all that, there's still these little operational quirks that happen, where, even if you know exactly where it is, you don't know what's about to happen or what the status is of things. Perfect example is, I know it's in the terminal, but I don't know if it's actually available for pickup, because I don't know if it's stacked at the ground and there's three containers on top of it, or if it's at the top and I can just get a crane to pull it off and put it on top of a truck, or if maybe the containers there, but according to US Customs, my paperwork was messed up, so there's a hold, and I'm not allowed to go pick it up. So there's all these other data sources that go into it that's not just about where it is, but about all these other statuses that really matter, that help inform what you can do and then subsequently, when it's going to get to the place that it needs to go. So is this almost a, you know, an issue at the port level, where they just have so many different moving parts, you know, per se, you know, literally and figuratively. Is it because they have all of these moving pieces and all these individual players that everybody kind of acts as if they're in their own silo? Yeah, that's, that's a part of it, and it's everybody has their own part to play. And so the real, ideal answer is, there's some third party that is the source of truth, quote, unquote, that takes data from all these places and makes it because you know the terminal themselves, where the container is going to be dropped off by a ship relies on the ocean carrier to tell them when the vessel is going to get there. But then, once it gets close, the ocean carrier, who's leasing the vessel or owns the vessel, relies on the terminal to tell them when the vessel actually docked and when the container got pulled off. And then they also rely on US Customs to tell them, Hey, are we allowed to give the container out? Because there's all these different people that are required to, like, you know, give their different inputs that somewhere along the chain to where it all flows seamlessly, and that's why there's that weird kind of black box of what's the source of truth? Because there isn't really one person that is the source of truth. There's all these different people that are interacting at different points in the journey. So how did you, and I believe you're, you're one of the co founders, is that, right? I think my title is not technically co founder. I was like the first employee. So that's, that's from what I understand, I heard, you know, that the business sort of started out with, you know, post it notes. Or maybe you can explain just how did the business start out? Because I would imagine that you're looking at all of these different data points, and it's like, what the heck, how do you choose to, you know, start a business that centers around those different data points? So you heard the post it note story that's funny. So Austin, our CEO. He originally started Gnosis as consulting. We were working here in Charleston with a freight forwarder. And the idea was basically to take his knowledge of supply chain and data science. He studied at Auburn University, just like I did, and just to take his knowledge of supply chain and data science to come in and help the freight forwarder automate processes. And it was literally the first product we built. Was hey, email is coming into an inbox, and we're going to read the email with code, and we're going to say, Hey, this looks like it belongs to this account, so we're going to send it to this account manager, and then they're going to take that invoice and pay it, or they're going to look at the invoice and see or we're going to take this and send the invoice to accounting. There's all these kind of things that we were doing. Because all they were doing at the current point was they had an employee that was looking at the emails and like, Oh, this is so and so's responsibility, and forwarding it off to them. So it really started as that. And the post it note story is the first time I came to Charleston. I was about to move to Chicago and go work in Chicago trading options. So I was going to be a quant trader, and Austin convinced me to come hang out with him in Charleston. And I went in and he had like, a fold up table, like one that you buy at Walmart, and just sticky notes all over the wall of like, this is how, you know, customs gets clear. This is the different places that that wear a container changes hands and all that kind of stuff. And it was just like trying to map out this entire global supply chain process. And for whatever reason, I was like, Oh, this looks awesome. I definitely want to be a part of that. And then the rest is kind of history from there. And so you guys, when was that timeline? You know, how far along or how many years ago? Did did all of this start? Austin started the company in 2017 and I came and worked with him for an internship while I was in grad school. It was just me and him at the time in 2018 and so, how did it, how did, how's the company evolved since then? Because obviously we've had, you know, a lot of things happen since 2017 you know, just a few black swan events. No big deal. How did the company evolve through all of those different, you know, diff, I guess, Black Swan data points as well. It was a, it's a a lot, I try to try to explain to some people, it's like a lot of cases of imposter syndrome, over and over. And like imposter syndrome, like assuming that someone out there knows better than you like, if this, if this could be done, somebody would have done it. So it was. Lot of, oh, well, we just want to build software that helps this one niche, because someone definitely has good data, container tracking data out there that we can just buy or source and do it. And so it was like we solved this problem. We all right, the customer is asking us for more. We try to find somebody that does it. If there's not somebody in the market that does it, okay? Well, we can try to do it ourselves. And a whole lot of iterations of doing that over and over, even to the just tracking data itself. We had tried to source tracking data like the actual ocean carrier milestones of like when it gets loaded on a vessel, and those things from the ocean carriers through EDI messages. And then that wasn't good enough, so we went to a couple of different data providers in the space and tried those people out, and then it was always like, if the data is not good, our software doesn't work, because our software was so dependent on we need to know exactly when a container discharges and is available for a truck to pick it up, or what the ETAs are going to be to a really granular level, to where I think this was like early 2020, I told Austin, hey, I think we need to do this ourselves. I think it's a it's important enough to the software that we have, that we have our own container tracking data and we're in control of it. So we did it, iterated on it a whole bunch. We get feedback still to this day, every single day, about hey, we really think one of our customers, hey, I know that you guys are doing this, and this would be really cool if you could add this data point, because it's useful for this thing. And so our container data model is always changing, and if we were relying on other people for that, we wouldn't be able to make those changes and continuously improve what types of or, I guess, what modes or data points have been the most challenging to integrate or even start collecting. It's, I think, the most difficult part for any of the sources. So I'll say, like ocean carriers, terminals, the rail, the class one rail carriers themselves. So like the BNSF, Norfolk, Southern CSX, the people. And I'm just talking about North America for rail, of course. But the satellite data, the most difficult piece is understanding that each of them all has their own data model, their own, what they call milestones. So like, what you know, the rail carrier calls a loaded on rail date, or a discharge or whatever may not be what the ocean carrier is calling because maybe the rail carrier is discharging it here and then putting it back on the rail and going to a different spot. And so the hardest thing is to between the getting the data from all the different places, putting it into one giant data model that makes sense for what our customers care about, and that's where we have really put a whole lot of effort into, because that's what our customers pay us for. That's what they want, is for to get really, really good data, but also to get it in a way that they can use it. And so when you're collecting this data, I'm I'm curious, how do you know if it's good data or, you know, just the data sucks. I mean, there's a whole lot of validation that goes into it. There's a whole lot of redundancy. And so people ask us about that, and, you know, one of the things that we do is you can get some redundancy from some of those sources. In the example I just gave on a rail carrier giving a milestone, or when a container is getting unloaded from a vessel at a terminal. You can get that data point from the terminal, or you can get it from the ocean carrier, and most of the time, what's happening behind the scenes is the terminal is sending that data point to the ocean carrier, and then the ocean carrier is sending it to us, for example, right? And so if we get it directly from the terminal, then that kind of eliminates the middleman, eliminates the three or four hours of latency that you have to wait for the data point to get back and forth. And so there is an element of we try to get it from multiple sources and do some verification, and then we have a whole bunch of the Super buzzwordy machine learning, AI, whatever you want to call it, that looks for the things that could be wrong, looks for the anomalies, and flags it and tells us that there's something wrong there, and then fixes it, hopefully, on its own. And so how do you with say, you know, a typical customer, they what does the onboarding experience look like for them? You know what? What is? What are they looking at within the Gnosis platform that makes them be able to take action? So we, this is something we really pride ourselves on. And, you know, you hear this, there's all these memes about, oh, I have a database management system where I manage my supply chain. And then it's really just excel like, it's like, people are just using Excel and emailing looking at you f1 people are just using Excel sheets and emailing them back and forth, right? And so we, you know, instead of being like, oh, Excel is dead. Like, that's dumb for people to do that, we kind of tried to make our platform look like Excel on every single demo that that Michael does our CRO Michael rents every single demo he does. He says, if you know how to use Excel, you know how to use Gnosis. And it's because we made the tables look like Excel. They're literally conditioned formatted of it's red if your containers in danger of getting demurrage. It's yellow if it's going to be a couple of days away. So you got to kind of watch it. And so there's all these things that we've done to kind of mirror how we were talking to people that were running their supply chains, so that there wouldn't be this huge change management, right? It's like, Oh, I was doing this stuff in Excel, and I had this cool. Pivot table that I use every day, and now I gotta go into this weird system and click a bunch of different buttons and do it. So that's the one piece of it is to kind of mirror what they're already doing. And the second is to take the data from where they're at, and then we have engineers. Every single one person that's a customer, or every single account that is a customer of ours has an engineer and Gnosis, that is their account manager that writes code that takes like, hey, they're like, Hey, we get this report every day from the ocean care that tells us what shipments have been booked. And so we write the code to take that report and turn it into Gnosis, like we write code that takes the container number and the bill of lading number, or whatever it is, and puts it in our platform for them, instead of going through the six weeks of an EDI implementation or figuring out whenever their IT resources are going to be ready six months from now to do a full on API integration with SAP, or whatever it's going to be, we want to do it as easily and quickly as possible, and make it look like what they're doing to foster that adoption, so that they don't have to wait for all these things to happen, so that they can get the value out of what we're doing. And so as you maybe the beforehand, before someone becomes a customer, if they're used to sort of, you know, if it's not, you know that ain't broke, don't fix it, type mindset. How do you, as a shipper, as a customer, know that you're working inefficiently, that your your data is probably inaccurate or it has some latency issues. How do you know? And what are maybe you kind of struggling with before you reach out to say, you know, sort of a become a customer of Gnosis. I think a common theme that we see is if people are spending all their time looking for the problems instead of solving them. That's the first red flag we have so many of our customers that their job before Gnosis was, hey, I got to figure out what might be costing us money. Like, you know, there's, oh, we had demurrage or per diem, detention, whatever you want to call it, last year, because containers got stuck at the port, and they have these Excel spreadsheets where they're sorting by the last free day and understanding and doing all these things. If people are spending their time doing that instead of acting on it, like sending a trucker to go pick it up or understand telling the warehouse, hey, this last three days tomorrow, we need to get this unloaded and sent back ASAP. If that's what they're spending time on understanding where that is. That's the red flag. That's where we come in. Is instead, because we we think there's things that computers are good at solving and things that humans are good at doing, we're not trying to replace employees. Our our marketing pitch is never, hey, you can, you know, you had 10 people doing this. Now you have five. You save money. It's take the people that were previously looking for all these things. Let Gnosis find it and then let them act on it. Let them be the Hey, according to Gnosis, we gotta do this and this, call somebody, send an email, do whatever it is, to act on it and then make their supply chain run better. So how, I guess you know, from a from Gnosis perspective, how are you creating that single source of truth with all of these different moving parts within the silos of the supply chain, but then also all of these different tech stacks that all of these companies are using. Is, how does that work? How does that function? We've done a our engineers are super smart. Like, I'll like, say, like we but really, like, I'm the dumbest engineer. I just, I just know how to, like, talk to the guys, and they're super smart, and they tell me how to do things right. I'm like, Hey, I think we should do this. Is this possible? But we've done a really good job. I think of meeting everybody where they're at, you know, I'm talking I'm talking to you about, we meet the customer where they're at, which is working in an Excel spreadsheet, and we try to mimic that. But if a terminal and add terminal examples. Off the top of my head, if they have an API, awesome. It's like, hey, we know how to work with an API. We can make that work. If they can send us an Excel file once every couple hours in this with a CSV that comes through a FTP transfer or something, no problem, right? There's we've seen everything from sending us an email report every hour, SFTP, Edi API. Even to at the far end of the spectrum is like, there's someone that is typing a message and it's text, we're like, okay, just send that to us, and then we use AI to parse it and get the data out of it, and then put it in the database. So like, if someone sends an email and says, hey, the last free day is tomorrow, April 18 or April 19. I guess then we will take that out and put the last three days, April 19 in the platform, so that somebody can see that. That's what's happened. Now I've heard you mention demurrage and detention a few times, maybe for folks who aren't completely familiar with those phrases. What are they? Why are they a problem? And do you think that they'll ever be fully eradicated from, I guess, a visit, a visibility standpoint, so demurrage, being the time that a container is available for pickup has been unloaded from a if there's maybe there's a part where it gets on a train and there's a railway. At the end. And that in that case, the merge will start when it's at the rail yard after it gets off the train and it's ready to be picked up. If they at a rail yard, sometimes they have 24 hours, 48 hours. It's a shorter time slot where, if they don't send a truck to go pick up that container, they're going to start getting charged, basically, for storage, you know, for the real estate, right? And so in that case, if they don't go pick it up at a certain time, then they start getting charged. Sometimes it's$300 a day, sometimes it's 50 bucks a day, whatever it is. And if they don't go pick that container up, they're getting charged for leaving their container there on the opposite end. I said detention. Some people call it per diem. That's when you take the container away. So a truck picks it up and brings it out, and then you keep it out for too long, and that's the ocean carrier, in that instance, charging you for keeping their equipment out. Because if you keep it out for 30 days, that's 30 days that they could have been using it to ship something from, you know, they fill it full of something else, and somebody else paying money to ship that container somewhere else in the world. So it's almost like container leasing. Yeah, that sounds like a whole, like separate business, or probably a revenue line item, which is probably different incentives for maybe some of these companies, exactly. And you asked the question about, you know, will it ever be eradicated? And there's, there's times when it's worse, then there's times when it's better. I think right now, we're, we've seen across our book of business, and talking to people and everything's the problem still exists, but it's not near the levels of money and time and things that it was in the middle of the pandemic. So whenever it was really hard to get trucks to go pick things up, and you had no room in the warehouses, because you're just picking containers up, unloading them and trying to ship out goods all the time, the ports were used as storage, almost strategically to a certain point. So instead of going to pick up a container and bring it to your warehouse, you didn't have anywhere to put it, so you would leave it and be like, what, $300 a day. That's fine, because we can afford that for now, because we're selling everything in our warehouse, we're going to wait till we have room to put it somewhere and then so that's it. I don't think it'll ever be fully eradicated. The penalties, while sometimes they seem crazy, I think they're there for a reason to kind of keep things going and incentivize the movements of things in the supply chain. But I think for if I'm understanding correctly, I you know the the main plight for a lot of the the Gnosis customers is that they want to avoid some of those unnecessary fees, and your software can help them on a daily basis to sort of find those anomalies, or I think you called it like, you know, exception management, find those different moments so you can avoid those extra penalties. Is that accurate? That's right. And sometimes it's not avoiding them, sometimes it's optimizing them. And I'll use the same example of like, where, you know, we have, so we have five containers at the port, and I only have room to pick up three. And maybe it's like, I know the way that my supply chain works. I'm going to use the time at the port of storage. This is a, hopefully, this is nobody's supply chain strategy is to incur to merge all the time. But in cases where it happens, maybe we tell them, hey, if you pick up these three, then the other two are going to cost you $50 a day. But if you would have picked up the wrong ones, they cost you $400 a day. And so there is a little bit of optimization and method to the madness. For not just like, oh, we want to avoid it at all times, which is the ideal goal, right? But sometimes it's smarter to incur $50 here and there than it is to oh, I need to Bobtail a container. So bobtails. I need to send a trucker with nothing. One way to pick up a container and deliver it, because that's a bunch of empty miles. So so maybe there's a better way to maybe pay 50 bucks to leave it at the port one day in order to ensure there's no empty miles. Is there any part of the shipment process? Because it it feels like, you know, over the last handful of years that we have found a way to make data points of every part of the logistics process. But I'm curious if the if there's any aspect of the shipment journey from source to porch that is will never have full visibility, or would be really challenging to get that full visibility, the hardest part and the thing that we do our best to solve is connecting the dots between what is inside the container and what the container is itself. And so you you're bringing up the example of, like, getting it all the way to, like, the customer's door. Like, if you know, I order a t shirt, right? Or I order masters tickets, or whatever we talked about the Masters earlier, if I order something to my door, a t shirt, example, works better for this one. If it came in a container from Asia to the US, I can track everything by that container. I know where the container what container it's in, and so therefore, I try the container. But then when it gets to a distribution center and it gets unloaded, there's a separate database and a separate tracking identifier and all these things that is important to track it from the time inside that distribution center, maybe to a store or maybe to another distribution center, and then it gets picked up by United States Postal Service or FedEx, or whatever it is to be delivered to my house. And so the hardest part of putting all these things together is knowing how to organize this database. Is with this one, with this one, in order to connect them all and give you that full like it was at the factory. And then it went here, and then I went here, and then it went to this, just all the way to the end. We're getting there. We do that for a lot of our customers, but it is difficult to understand and index and do all the different database V lookups, right? I mean thing Excel, it's a bunch of V lookups from this one to this one to this one, to get that full end to end picture. Is there any kind of effort within the industry, just as a whole? Maybe you guys are trying to do this, but any kind of like data standardization, you know, across the globe, or across maybe just the United States, like starting very small, maybe moving outwards. Is there any kind of issues, or not issues, but efforts being made in that regard, there are, and I think, you know, I mentioned how everybody has their own systems earlier. Everybody has their own data model, their own way of doing things. So if there was standardization in the industry that everybody adhered to, that would be easier. And I think there are efforts being made. The dcsa, the digital container shipping Association, has done a good job of working with the ocean carriers themselves. Of hey, you know, if we get all the ocean carriers to follow this data model and their APIs and their data exchange that they're participating in, then it makes it easier for both individual shippers software companies like us, freight forwarders and so on, to digest that data and do things with it even. And they're, they're doing great work, and I'm excited. I know the guys there, and I've talked with them a bunch, and hope to continue to give feedback and help them. But for us, you know, we kind of take that data model and take what we get from all these places and and mold it to what we think our customer cares about. So I mentioned, like we we show our customers the things that are important. So we maybe, in the example that we always talk about is if they're if a containers go into California, and then it's getting on a rail and going to Chicago, we're not going to give you, like, the last free day and all this stuff in California, because it doesn't matter to our customer. What they care about is when is it going to get to Chicago, and whenever it's in Chicago, when can I pick it up? When's the last like, how am I going to get charged for things? And so there's all this business logic that we put around the data points that we get from all these people to show our customers what they really care about. And so it's, you know, I mentioned how we were pretty naive, and then we've gained more and more experience and iterated over time to where we add new data points and subtract and change the data model over time, because we're getting that continuous feedback from our customers on what they really care about and how they're going to use it. Are, you see, are you able to, you know, with your customers? I imagine that you're collecting a lot of their data as well. Is there any kind of, sort of a global spreadsheet that you're looking at with everybody's data, that you can kind of notice trends that are happening, maybe before your customers do definitely. And the cool thing that that our engineers, again, not me, I'm the dumb guy over there, but the engineers that have figured this out, it's super smart for taking you know, there's everybody's supply chain is different, right? So if you just throw every single container in a giant spreadsheet and be like, I want to make predictions, it works, but there's a part of it that's unique to every person. So trying to an example, I always use this trying to predict someone who moves five containers a year, because they ship them to directly to their store. It's like a small furniture company, and they do something like that, trying to use their data to predict how Amazon's containers are going to flow. Doesn't exactly match, because Amazon that does all these containers might have a totally different priority, where they may lead. They have better contracts. They leave containers at the port for longer. They keep them away from the port for longer, and so their supply chain works differently. So what our engineers have done is kind of, we have like, a ubiquitous data model for everything. It's like every container that flows from Shanghai to Los Angeles is on these big vessels, and we know the route that it's taking and the stops that the vessels making along the way. So we can make predictions for, you know, say 80% of it, and then the last 20% is at the customer level. So that person that moves five containers, as soon as we get some of their containers in our system, and we understand how long they usually leave at the port and how long they're keeping it away, and how their supply chain works, you can kind of take both models and add them together to get something that makes sense for them, that almost sounds very similar to, you know, what's going on with like, the large language models, where they're probably going to reach a certain level of parity soon, and they have a, you know, they're all pulling in there. There's certain amount of data, but then what's really going to push it over the edge for the end user is using your own data. In addition to that, that level of parity. I doubt we've reached a level of parity when it comes to logistics data, but that's what it kind of sounds like, right? Yeah. I mean, giving it context, right is like you can the large language models that we're all used to, was trained on so much, you know, YouTube data and publicly available information on the internet. And so much texts and languages and all those kind of things, right? And so in order to make it super intelligent about, say, Gnosis, for eight we give it, hey, read our website or look at our API documentation and do those things. And then the second you give it more of that context, it gets better. It's the same idea for what we do with our customers, where, if we, you know, tell it we the large language model that we have in Gnosis is equivalent to, like, tracking every container from port to port, or port to rail, or whatever it is, right, and then the stuff that happens, where it's delivering to a distribution and something is the context that it needs to make those predictions outside of the normal model. How, speaking from, like, a, you know, a global scale, how, with all the data that you guys have access to, how does the US compare from, you know, Port efficiency versus the rest of the world? Are we doing okay? Are we doing not as good? You know, what? What does that comparison level look like? I'll speak to some positives. I'll say from a positive perspective there, you know. And people ask me, like, what's my favorite port and all those kind of things. I think there's some big strides being made at some places in the US. I think the Port of Virginia is a great example. They do a great job embracing some new technology and talking with technology companies and doing things and their efficiency and all these kind of things. It shows, you know, there's these the global like Port efficiency rankings and things. You know, there's like, 353 ports in the world, or something like that. And some of our larger ports in the US are at the very bottom. And then you look at Port of Virginia, and they're like, you know, not at the top 10 or top 20, but pretty far up there for and they're continuing to gain strides in that, because they're embracing technology and doing some cool things. You know, I don't, I don't want to speak to any of the negatives. There's a lot of the regulatory stuff and all that that comes with, with why, maybe we're not at some certain places in the US, but overall, there's, there's some people that are doing some great things, and I hope it continues. Yeah, I think I was listening to your interview with Mike Lombard with Yes, great interview with him. And you were telling a story about how you went to Spain and you hiked up to the top of this mountain, and then you looked over at the port, and it was almost a little eerie, because there was nobody working, and it was all automated. That was my wife loved that we were in Spain. I found a way to incorporate containers, and was like, Oh, my God, there, that's APM Barcelona. There's great. We had, like, done this cute hike, and I topped it off. I'm like, Oh, look at that terminal. So yeah, but I mean, it is and that that's, uh, I think there's a lot of opportunity for improving that. And I, you know, the whole idea that people talk about around AI and automation and things is, like I said about Gnosis, the goal is not to replace people's jobs, right? Is, and I know that that's the fear and a lot of these things, that's never the goal. The goal is to replace the stuff that people don't really want to do. Right? Is the monotonous like moving a container from, you know, Spot A to spot D, or doing things like that. That's where I feel like we can take advantage of AI and automation and those kind of things so that they can do more creative work and do cooler things and make decisions and do that. That's I feel like the goal. And I think that for all the things that the US is good at I think that's one that we're a little bit behind. Yeah, I would agree. Because I, you know, I've had conversations with people who work at ports, you know, all across the globe. And it's one of those things where it's, you have to balance the technology with the human element. And even though it's really cool to see, like a fully automated port, you know, that's, you know, there's a lot of job loss there, too. And you know, how do you balance what that looks like? And I definitely see the efficiency gains, but I do wonder how we can still keep the human involved at that high level like you were talking about. And so I think there's definitely a balance to strike, and I don't know that the US has sort of reached that balance. But I would probably counter that own point with each port kind of has their own decision to make in that regard. So I think that that's that's a little bit cooler of a feature for us based ports, is that they can choose their destiny. They can choose to embrace jobs or technology and maybe a little bit of both. That's right. And I'll, you know, make the parallel of whenever set the notices examples we come in. Our goal is to help people not have to go check 100 different websites to understand what's going on with their containers. The idea is then not to fire that person, but it's to let them think about what's next like, you know, maybe the fact that they didn't have to spend four hours figuring out where all their stuff was. That means they can call their customer and ask how they could do more. You know, let's say, hey, like, all your stuff's ready. But, you know, I saw that you're struggling in Vietnam, and we have this supplier. We know there's all kinds of different things that could just be it's better suited for their time. They could bring more value to the business than them just doing them anonymous work of day to day. Where is this and putting it into their system. Now, we talked a little bit about the Spain story. Do you have any other stories that you've kind of learned from being involved in the data so heavily for, you know, a handful of years now, you mentioned Vietnam just now? Is there anything kind of surprising that stands out to you Data Wise, from just global trade in general. I mean, just at a holistic level, it continues to surprise me every day that, like the imposter syndrome I talked about is like, there really isn't, like some behind the scenes, like all seeing data source that we just don't have access to, and they're not telling us about. And I'll say that from the perspective of you know, it's not like the big, bad ocean carrier has a bunch of data that we don't, and therefore they're charging us money. And that's that, like they're just taking advantage of people. It's really that the data just doesn't exist. There's not like, a some place that's figured it all out, and then they're just hiding it from everybody because they want to make money off of it. It's just that everybody's kind of in this together. And I mean, it's talking to all different parties. And you know, we work at Gnosis, we work with the ocean carriers, we work with the terminals and ports themselves. We talk to the rail carriers, we talk to the shippers, the drayage carriers, the freight forwarders. We've talked to every single person that's somewhere along the way, and they all kind of have similar questions. And it's all asking about, where does this data point come from? How can we do this better? It's different data points because, you know, everybody relies on somebody else to get certain ones, but no one has a perfect answer. There's not like some place that has it behind the scenes that we wish we could all grab it. They're all trying to coordinate together and figure it out, which has been super cool for us to start to gain a little confidence when we're talking to the ocean carriers or talking to the ports or talking to shippers and play pool, is like we've talked to everybody we feel like, and we keep improving our data model based on all the different people that are telling us about it. Within the models, does it exist to sort of omit some of these black swan events, like, is that? Is that a, maybe a thing like, you know, I would imagine, like, you know, removing Amazon from your data set, obviously, you're going to see some different fluctuations in that regard. You're going to remove a lot of, a lot of shipments from that regard. But I'm almost curious if, like, if it's more valuable to remove some of the bigger players, or the bigger black swan events in order to make that data a little bit more actionable for customers? Or do they find it's better to look at it for more of a holistic view? I think, you know, we're in order to make predictions and do things. We're eliminating the outliers all the time. You know, in order to get to that, like best estimation, we're taking stuff out and making predictions based on whatever we have best available. Was talking to somebody yesterday, and we were talking about, hey, this eta was pushed back by 40 days. You know? Why was Gnosis wrong? And I have this conversation, and I am sitting there, and I'm we're looking at it, and it's like, man, we made this eta prediction because we thought it was gonna follow this path, right and and then we go dig into the data. And the container had gotten unloaded in Panama at a terminal, and it was supposed to, you know, get on another ship, like, three days later, but it didn't, and it just sat in Panama for 40 days, or whatever it was. And so that, like, there's these things that happen, where it's just operational or, I don't know, and there's maybe a way we could have predicted that it would have been delayed a little bit, but there's no way for us to, like, there's all these like outlier things that happen. There's no way to really predict that. It would have just sat there for 40 days. It's just, like, an operational thing. Maybe they just forgot about it, right? It's like, there's stuff that happens that if you try to predict everything, then you'll predict nothing, right? And so trying, trying to get the good data model that I can talk to our customers and say, Hey, we can tell you with 90 95% certainty that it's going to get there by this date. That's hopefully good enough. And then we continue to try to get that better and better all the time. But then in the situation that happens, all we did is we've made it red at the top. We're like, hey, something's happening here. And that's where they started to drill into it. And they go to their provider and say, what's happening? Why? Why did you leave my container at a trans shipment terminal for 40 days? Yeah, I imagine it probably falls into some of those examples like you were talking about earlier, where you want to manage the exceptions instead of the rule of the, you know, I would imagine that most shipments are error free or hassle free. Is that a safe assumption is the majority of our shipments hassle free? Yeah, and especially now you know that, like, there's the the craziness that happened with the Suez Canal, the stuff that happened in the Panama Canal with droughts, but and then, you know, go back to COVID Whenever ships were hanging outside LA for a month or longer. You know, that stuff kind of has subsided a little bit. I know that the Suez Canal still is an issue, but the ships that are going around the Cape, and they have their normal transit times and stuff, there's not a whole lot of craziness happening, right? And so in that case, you know, you can get to we have this eta prediction, and maybe there's a day. That slips here or there based on how long it stays at any individual terminal or stop along the way. But for the most part, things are working pretty well. What about this is going to be a completely random comment. What about like piracy? Is that still, you know, obviously, you know, there's things going on there. There's issues going on within the Suez Canal. You know as Somali pirates were one of those things that you heard about for a long time. You don't really hear a lot about them anymore. But what about finding those kinds of outliers it? You know, I was watching a video, YouTube video last night. It made me think of this, but like all the Pirates of the 1700s 1800s that were in the the Caribbean, and does piracy still exist in sort of modern ocean shipping? I'm sure it does. I'm sure that there are instances of piracy. You know, I think going around the Cape of Good Hope that was one of the concerns is that there would be more of the piracy and things that happened down there. I'll say the thing that we've seen more is theft on the domestic level, so containers in rail yards, either in LA Long Beach or somewhere between LA and Memphis or Chicago getting broken into that we've had a lot more questions about than the piracy on the ocean side, which is Yeah, so and people ask us about that. You know? How can you help us prevent it? And or do you know? How can you help us know when this happens? And it's a super interesting conversation to have to understand, and people are telling us, yeah, it's, you know, X percent of their shipments get broken into when it's sitting in a rail yard. It's crazy, yeah, so how are you advising them to sort of get around that just, you know, move their shipments from, you know, these, I guess, high crime areas. We collect all the data of when it happens and tell we give them the insight to, hey, well, whenever you move it on X route between these two places with this carrier, whatever it is, it seems like it's more likely to be broken into. And then, you know, there's, I don't know, short of us putting a security guard out there that I'm not sure what, I don't know, what else we could do, right? So we're continuously evaluating that. That's always a super interesting conversation to have. Yeah, I've had conversations, you know, with it, you know, insurance folks who provide insurance to, you know, the industry, and that they have software that just tracks those high crime areas tries to, you know, help them avoid it, and geo fencing, and there's all kinds of technology that's, you know, come into logistics that is really fascinating with some of these problems that they're trying to solve. And I guess, in that, that similar vein, where do you see, you know, with this tech adoption going, you know, it feels like all companies now know the importance of technology adopting, you know, evolving using, you know, AI machine learning, however you want to phrase it, where do you see things evolving? And I don't want to say five years. I feel like that's impossible to determine, but maybe the next couple of years, sure, and I'll double down on what I was kind of saying at the beginning. And I know we've talked a ton about data and ETAs and predictions and those kind of things, and Gnosis does that. I feel like we feel like we're the best at it. We do it a whole lot. But the stuff that gets us super excited, the stuff that our customers get really excited about, is all the execution stuff that's in parallel to that. So if you get the data right, then you can start to do the cool things. And like a couple examples, is like the financial piece. So auditing invoices, making sure that you're getting charged the ocean freight rate, that you should the drayage rate, that you should, that you the contracts that you've agreed on, that if we have all the data, then what are the things that you can do with that data? You can make sure that everything else matches, right? So the auditing piece, the actual execution of, you know, I mentioned, like, if you figure out something is in danger, to send an email to somebody, well, that's something we can automate, right? It's like to make sure that every container that is coming in has somebody that's responsible for it, to send a delivery order right to a drayage carrier or so on. We, we've, we've built, and we continue to build, the tools to allow people to do their job in Gnosis, instead of just using it as, like a business intelligence tool, like, Where's my stuff? But actually, okay, I know where all my stuff is, and I know I have to do these things, but I can get noses to help me do those things. Yeah, that's, that's such a that's another sound bite that we'll probably use in a future clip, you know, to promote this episode, last couple questions here. And, you know, this is just a curiosity one, because I, you know, I live in Jacksonville, very close range to Savannah sport. You know, we have Jack sport here. Of course, there's a little rivalry between Jack's port and Savannah, and I was curious, of you know, is that a similar feeling on the Charleston side of things, that there's a little rivalry with Savannah? Yeah, of course, I think so, you know, Savannah has been crushing it the past couple years. They're a lot bigger than Charleston and Jacksonville, and they've been doing a great job. We have relationships in both places and kind of, you know, they're so I'll speak to the port piece. There's definitely the city rivalry. You know, we think Charles. Way better than Savannah. And we'll, we'll argue that they're our little brother, little sister, whatever, all the time. But anyway, on the on the port side, I think that the the port leadership, works super well together. And although they have, like, slightly different philosophies, and there is a little bit of that rivalry, they do a great job of coordinating and talking with each other and sharing strategy and those kind of things. Good example is that was funny, whenever all the congestion hit and COVID and Savannah got super backed up, a lot of people diverted and went to Charleston. Well, the problem was everybody decided to divert and go to Charleston. So there was like, a like, we have a chart that was like, you know, delays in Savannah, delays in Charleston, and it like crossed, and it was like, delays in Charleston went up, and delays in Savannah went down because they did such a good job of coordinating that everybody switched, and then it just happened here. Well, I think that's we're going to bring this episode full circle, because we started off by talking about Southern charm. The Charleston series lasted, is still going on the savannah one. However, it only lasted a couple of seasons, maybe even one season. So I think we have another comparison there, too, the city the port and editions of southern charm. So Jake, this is a really fun conversation. Anything that you feel is important to mention that we haven't already talked about. I think whenever I talk on a podcast or anything, I'm always super self conscious that I give credit to the employees at Gnosis. I think that, you know, I mentioned a couple of times on this podcast, like, Hey, I'm the dumb guy, but I I really mean it, that the we are super unique in a software company where everybody that works in Gnosis is in this building, and so, like, I'm in my office right now, and everybody that works here is right outside. So they're in their offices, and they all, you know, cubicles and all those kind of things. The people that work here come to the office every day. They open their laptop, and they're solving engineering problems for our customers, and they're doing that on a global scale, you know, from 8am to 6pm eastern time, but then also answering emails at 11pm and 3am for all of our customers are all over the world. So I'd be remiss if I didn't take a chance to thank them and just like give a shout out to the guys that work here, that they're all doing a really incredible job. That's that's super interesting to hear, because a lot of folks are, you know, like, oh, we want, you know, hybrid. We want work from home. But I mean, obviously you guys are doing some some great things with an employee base that's all in house. We, yeah, we, I say we, Austin and myself really have doubled down on that since the beginning of the you know. We, it's definitely hurts us sometimes where we can't hire some of the talent we want that maybe lives in San Francisco or New York or wherever it is. But we, there's a siren going by outside, right? Facts, but we, we think that it hurts sometimes in the short term, but in the long term, we feel very strongly in the culture and everybody being here and being able to collaborate and those things has given us a huge advantage when we've been building products and making changes and doing things that's awesome to hear. Yeah, it's definitely you don't hear that perspective often now, but I think, you know, a little bit of the tide is turning for for a lot of these companies that are requiring folks to come back into the office, that there is some synergy. There is some, you know, brainstorming that you can take advantage of if you're working together as a unit, versus kind of feeling like you're working in your own individual silo, which is, you know, has its own challenges. And I think it kind of happened where that COVID, like going remote and doing those things we were, we went, you know, remote whenever everything first happened. And at that time, it was probably seven of us that were working at Gnosis at the time, like 2020, when it was really, really really bad. And then we looked up one day and we had all, like, been in the same pod, and we're, like, eating lunch at someone's house, and Austin called me. He was, Are you kidding me, you guys, you guys are all together, and we, like, went remote so that nobody would be together. And, like, cause like, like, spread anything. So we're like, oh, well, we were all like, together all the time anyway, we might as well just keep working together. So it was a, it was a weird, like, realization that we were small enough at the time and we were all hanging out anyway, that, man, we could have just been doing this the whole time, right? And I think that that's what, that's the mindset that a lot of companies took, is, you know, they would rather, you know, be with their their people, and be within the group that they're always around anyway, so why not, you know, get some work done and, you know, make some money at the same time. Exactly. All right, cool. Well, Jake, this was awesome conversation. Where can I send folks who want to learn more, who want to, you know, upgrade their their visibility options. Connect with you on LinkedIn. Where can I send them? Yeah, LinkedIn, of course, I'm, I'm always on LinkedIn and posting dumb things on there all the time. Follow, following read Lucille example to try to post stuff on there all the time. Yeah, LinkedIn, Gnosis, free, Comm, our website, I think we have a form that you can go and fill out there, and somebody will be in touch with you. But in. Anybody can reach out to me directly. You know, my title is CTO, and that's like a technical title, but I do a whole lot of sales and talking to customers, and I just love nerding out on all this stuff, so I'm happy to talk to anybody Awesome. Well, we will make sure to put all of that in the show notes to make it easy for folks. But again, thank you for the conversation and the insight that this was a fun one. Thanks. Blythe, you. I hope you enjoyed this episode of everything is logistics, a podcast for the thinkers in freight, telling the stories behind how your favorite stuff and people get from point A to B. Subscribe to the show. Sign up for our newsletter and follow our socials over at everything is logistics.com and in addition to the podcast, I also wanted to let you all know about another company I operate, and that's digital dispatch, where we help you build a better website. Now, a lot of the times, we hand this task of building a new website or refreshing a current one off to a co worker's child, a neighbor down the street or a stranger around the world, where you probably spend more time explaining the freight industry than it takes to actually build the dang website. Well, that doesn't happen at Digital dispatch. We've been building online since 2009 but we're also early adopters of AI automation and other website tactics that help your company to be a central place, to pull in all of your social media posts, recruit new employees and give potential customers a glimpse into how you operate your business. Our new website builds start as low as$1,500 along with ongoing website management, maintenance and updates starting at $90 a month, plus some bonus freight marketing and sales content similar to what you hear on the podcast. You can watch a quick explainer video over on digital dispatch.io, just check out the pricing page once you arrive, and you can see how we can build your digital ecosystem on a strong foundation. Until then, I hope you enjoyed this episode. I'll see you all real soon and go jags. You.